Introduction
It is vital that education uses research in order to make evidence-informed decisions. Therefore, educators need to be able to understand and evaluate published research.
In this blog post I’m going to discuss research used in education. I will give an overview quantitative, qualitative and mixed-methods research. I will then evaluate a paper in my research area – educators’ use of generative AI.
Positions & Paradigms
In education research, it is important to consider the philosophical approach of the researcher to the research being undertaken. This can give us an understanding of the ontological and epistemological assumptions of the researcher, and should inform their approach to designing, conducting and analysing their research. Ontology is the philosophical concept of the study of being. The word comes from Greek, “ontos” – being, and “logia” – which is logical discourse. Antony Flew (1989) describes ontology as being “concerned with what there is.” In the context of education research Buckler & Moore (2023, p35) state that ontology is “a quest for understanding whether to work alone or to work with others.” That is, whether the nature of reality is objective and independent (a realist ontology) or socially constructed (relativist ontology). Epistemology, again from the Greek meaning the logical discourse of knowledge, asks the question “what is knowledge?” (Plato). It is a way of thinking about how we acquire knowledge and whether there are any limits to what can be known. In educational research we use epistemology to frame our thinking around the questions of how our knowledge is constructed. Braun & Clarke (2022) discuss epistemology as a method of evaluating the credibility, authenticity, and reliability of information. Axiology is the study of value – axia is Greek for value or worth. In philosophy axiology explores the values of ethics and aesthetics, investigating the nature of value and value judgements, essentially interrogating what is considered “good”. Within education research we use axiology to assess the value judgements or the value position of the research in order to place it in context. Buckler and Moore (2023) state that axiology “relates to what is ethical and unethical.”
There are several philosophical positions within research that we must consider: positivism, post-positivism, realism, interpretivism, and pragmatism. These define the research methodology and position it within a philosophical framework. Post-positivists view the world from a critical realist perspective. They believe that while there is an objective reality, our understanding of it is problematic and imperfect. Conversely, positivists see the world as an absolute reality – what can be observed is the ultimate reality, accessible through the senses. Within research, positivists would argue that the only legitimate knowledge is that which is gained through the scientific method. Realists believe that knowledge can exist independently of the knowledge held by an individual. It is a form of objectivism, which is the belief that reality exists independently of human perception or understanding. Interpretivists, on the other hand, focus on the subjective nature of reality, emphasising that knowledge and understanding come from interpreting human experience. They argue that reality is socially constructed and shaped by cultural, historical, and contextual factors. Interpretivism often relies on qualitative methodologies to explore the meaning, context, and perspectives of individuals. Pragmatism, meanwhile, takes a more flexible stance, asserting that the value of knowledge lies in its practical application and usefulness. This philosophical approach prioritises solving problems and addressing real-world concerns, blending methodologies as needed to achieve the research objectives. Pragmatism recognises that both objective and subjective perspectives can contribute meaningfully to knowledge creation. It is a rejection of the need place research and be constrained by a notional philosophical paradigm. Coe (2021) defines pragmatism as an anti-philosophical stance while Biesta (2020) regards pragmatism a rejection of paradigms altogether, seeing them as problematic.
It is important to consider these philosophical positions when reading research, as the position taken by a researcher helps to understand what perspective or influence that researcher has placed on their research question, data collection methods, and conclusions in the study. The philosophical positions inform the choice of methods used in research, and the methodological approach. Generally, there are qualitative and quantitative forms of research. However, some studies take a mixed-methods approach combining elements from both. Qualitative research tries to understand experiences through the perspective of the individual. Researchers using this method often collect data by asking questions of the participants and then develop themes from the resulting data. Braun & Clarke (2022) describe qualitative research as being focused on meaning and that it contributes to the “rich tapestry of understanding.” Quantitative research, however, is often based around the idea that there is a singular measurable truth – a realist ontology. In this type of research, the research is claimed to be objective and impartial, with no possibility of researcher bias impacting the data. Data collected in this way is numerical and usually subject to statistical analysis to test specific hypotheses. Robson (2014) states that quantitative research follows a scientific approach to research using the same principles as research undertaken in the natural sciences.
Checklists & Questions
To examine the usefulness of any given paper, it needs to undergo a critical appraisal. Greenhalgh (2019) suggests some questions that we should ask about a paper, namely:
- “Why was the study needed, and what was the research question?”
- “What was the research design?”
- “Was the research design appropriate to the question?”
We can use these questions to initially address a paper, considering the fundamental issues of the research, and whether the research has value. Once we have ascertained the relevance and value of the study, we can then move on to a more critical analysis. In the context of education research, there are several evaluative frameworks that can be employed. The Open University suggests the PROMPT framework (see table below) as a way of performing a critical analysis of literature.
Presentation | considers the clarity and professionalism |
Relevance | ensures the source aligns with the research question |
Objectivity | assesses the neutrality and bias within the paper |
Method | examines how the information was gathered and presented |
Provenance | investigating the credibility and expertise of the author or publisher |
Timeliness | checks how current and appropriate the source is |
The PROMPT framework. https://www5.open.ac.uk/library/help-and-support/advanced-evaluation-using-prompt
By using these criteria, the Open University suggests that we can make a reliable assessment of the quality, validity and usefulness of any given study.
Another source of frameworks to consider is CASP – the Critical Appraisal Skills Programme – as they provide downloadable checklists with prompts to critically evaluate various types of research. The checklists aim to assess the validity of the research by assessing the methodological rigour through appropriateness of design and the minimisation of bias. They check the results and findings to ensure the reliability of the results and if they are credible and measured appropriately. Finally, the checklists seek to evaluate the relevance of the study to local contexts, practitioners and policymakers. CASP aims to provide an accessible, standardised and consistent way of assessing research whilst being evidence-based and easy to use. Other frameworks can be found by searching literature, when necessary, to support evaluation of specific methodological approaches.
There is clearly some overlap between these methods; however, when used in conjunction with each other, we can assess a paper and identify areas where one method might not be as strong, allowing us to gain a broader insight into the research presented. Each framework has its own strengths: for example, Greenhalgh’s questions provide an initial assessment framework, whilst PROMPT offers a comprehensive evaluative structure to guide our analysis. Similarly, CASP is methodological and prompts the reviewer with questions to ensure a consistent analysis has been conducted. By combining these approaches, we can thoroughly evaluate the research in terms of rigour, validity, relevance, and implications for future research.
The Paper:
The paper I have chosen to analyse is Khlaif et al., (2024), “University teachers’ views on the adoption and integration of generative AI tools for student assessment in higher education.” This was published in Education Sciences, in October 2024. I have chosen this paper as it is similar to research I am considering for my own dissertation, being concerned with educator attitudes to AI use and whether the potential benefits are realised. The combination of its contemporary relevance, use of a mixed-methods methodology, and discussion of technology acceptance frameworks, which I also plan to employ in my own research, make this paper particularly interesting for analysis. A copy of the paper can be found here: https://doi.org/10.3390/educsci14101090.
Taking Greenhalgh’s (2019) questions first, I will now describe and then evaluate the paper.
“Why was the study needed, and what was the research question?”
The study is needed to improve understanding of the use of generative AI, especially around student assessment. Generative AI, which has been developing rapidly since early 2022, creates text in response to prompts. It has been seen as a threat to academic integrity, but also potentially useful to alleviate workload issues in education (Birtill & Birtill, 2024). There were three research questions, centred around the issue of how academics use generative AI to assess students, and understanding the factors that drive academics to use generative AI. The final sentence of the introduction states that the research aims to understand the decisions of instructors and professors who doubt the validity of generative AI in student assessment, in order to persuade them otherwise.
“What was the research design?”
The research adopts a mixed-methods approach, with a cross-sectional questionnaire including both open-ended and Likert-scale questions. The participants were self-identified generative AI early-adopter academics in Middle Eastern Universities. Structural equation modelling was used to analyse the quantitative aspects, and a thematic analysis was employed to understand the qualitative responses. The researchers used the extended unified theory of acceptance and use of technology (UTAUT2, Venkatesh 2012) to frame their analysis. This model uses concepts such as expectancy of technology, habit of use, and behavioural intentions, to predict actual use of a technology. This indicate that the research is aligned with a positivist approach, using a theoretical framework, and statistical analysis with a large sample size.
“Was the research design appropriate to the question?”
The research design was partially appropriate. To understand educators’ attitudes towards a new technology, it is sensible to employ an existing, theory-informed framework such as UTAUT2. The framework uses Likert-scale questions, which have been validated in a several different contexts. Indeed, the UTAUT2 has over 6,000 citations (Tamilmani et al., 2021). However, such a quantitative approach does suggest a realist ontology. This seems at odds with a research question that is investigating a socially constructed attitude. However, use of a qualitative component to supplement the quantitative analysis does at least partially address this. The researchers are not explicit about their ontological or epistemological positions, making it difficult to evaluate the appropriateness of the design and methodology they have adopted. However, the research is motivated by the need to persuade reluctant academics to use generative AI and so examining motivations in a group of early adopters seems inappropriate. More problematic, there is no discussion of ethics in the paper. It isn’t clear how consent was obtained from participants, and whether there was any ethical review of the research.
Having gained our bearings, and described the approach of the paper, we can now employ more detailed frameworks to continue our evaluation. Let’s first consider each research question in turn. The first question examines how academics use generative AI to assess their students and is addressed by a qualitative analysis of the open text answers. The CASP checklist for qualitative studies (CASP, n.d.) asks whether the results are valid, and what the results are, and if the results will help locally. Another approach to assessing qualitative analysis is the Braun and Clarke ‘Bingo’ card (see below). More seriously, Braun and Clarke have recently published their own reporting guidelines for qualitative research (Braun and Clarke, 2024). They discuss ‘big Q’ and ‘small q’ qualitative research. The results reported in Khalif et al., (2024) are certainly ‘small q’. There is no discussion of deeper meanings, or the contextual nature of the open text responses.
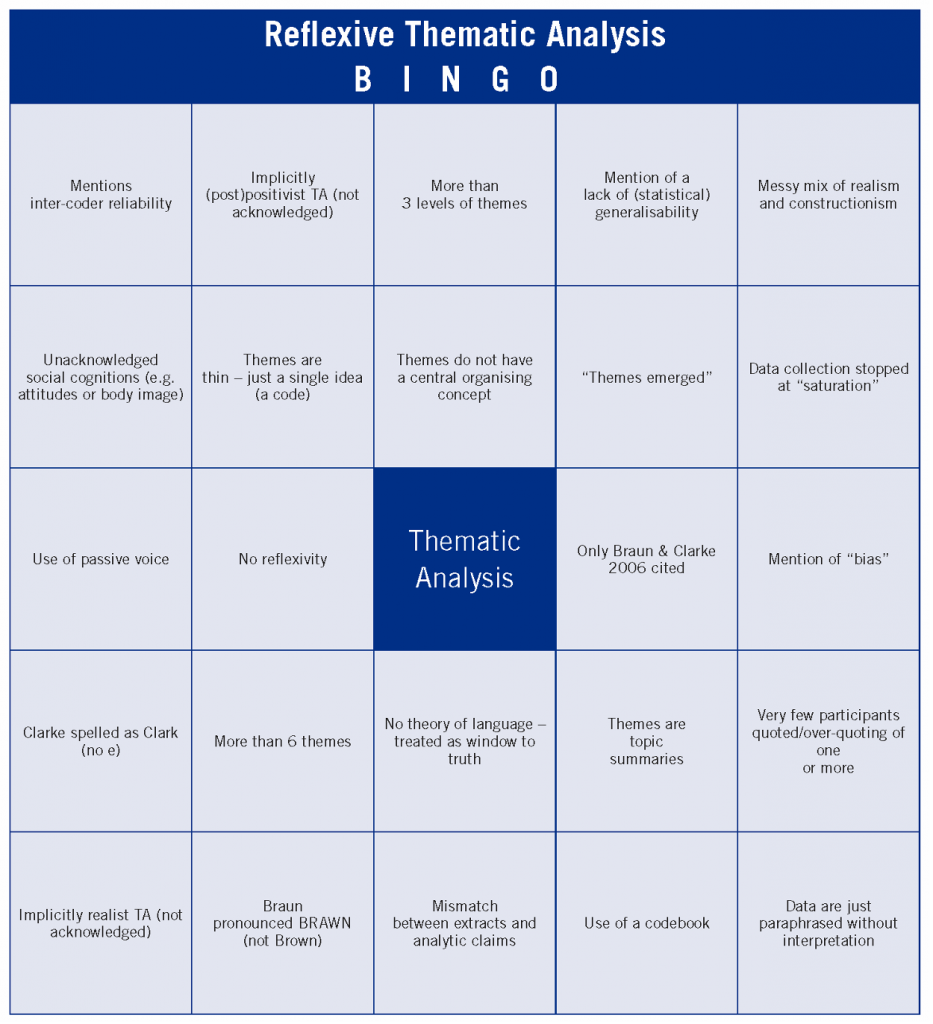
The paper claims to have used an inductive thematic analysis. However, an inductive thematic analysis would be used to develop ideas found in the data, finding patterns and themes in the data. However, the researchers here started with a theoretical framework of UTAUT2 and are using this model to test their thesis. In essence, they have conducted a deductive analysis where they want to confirm their hypothesis and have coded the responses to match the theoretical model. The paper suffers from what Braun & Clarke describe as positivism creep – the researchers are clearly valuing objectivity in the study and looking for an ultimate truth. The authors do not give any detailed description of their thematic coding, not even listing the six-stage process of Braun and Clarke. In their results, they report two themes, but these are closer to ‘topic summaries’ (Braun and Clarke, 2022) which describe the content of answers rather than the underlying themes, with data paraphrased or even quoted without interpretation. In addition, researchers using thematic analysis should state their positionality regarding their research position and consider how their paradigm influences the interpretation of the data. In this case, that has not been included.
From the qualitative analysis, the authors created a helpful flowchart showing the process that academics use to develop AI supported assessments. However, in the text, they seem to infer that this is a model that should be encouraged, rather than reflecting the process described by their participants: “The flowchart highlights the iterative nature of this process, encouraging educators to continually refine their methods” (p1090). This is confusing, and it isn’t clear how this flowchart relates to the qualitative data.
The second part of the qualitative analysis summarises three approaches to using generative AI in assessment. These align with other approaches that have been developed institutionally, for example The Russell Group guidelines (Russell Group, 2023) although the authors do not explicitly draw out these similarities. These approaches are banning generative AI, using it in a supportive capacity, and encouraging full use of generative AI in assessment. In this section, there is again little depth of analysis, and quotes are given with no interpretation.
The second research question, examining the factors that drive academics to use generative AI in assessment, is addressed by both qualitative and quantitative approaches. However, the qualitative section includes no quotes, and only a list of relevant factors.
The quantitative approach is to examine how well the UTAUT2 explains participants use of generative AI, using structural equation modelling (SEM). This is a statistical technique that allows the researcher to model the relationships between several constructs, and to understand which constructs predict each other (In’nami & Koizumi, 2013). Constructs are comprised of responses to items on the Likert questionnaire. I am not an expert in statistical analysis and feel unable to fully evaluate the claims made here. However, from looking at the data presented there are clear inconsistencies in the reporting and presentation of the results. This indicates a lack of precision and makes me question the validity of their claims.
The authors present the results of the SEM, but they don’t present any of the descriptive statistics. The ‘outcome’ measure they are trying to predict is ‘Use of generative AI’ on a 1-7 scale – but we are not told what the average use, or range of scores of their participants are. Given the inherent bias of including explicitly early adopters of AI in their sample this seems like a real omission.
In Table 5, the authors present the results of the SEM. P-values are provided, stating p=0.00. However, this isn’t appropriate, and p is never exactly equal to zero. All their hypotheses are supported in these statistics, with positive relationships between most of the constructs. These strong positive correlations between the constructs are also in table 4 – although this is only clear from reading the text, as the table caption is incomplete! As a non-expert, it seems slightly troubling that everything correlates so highly with each other. This is unusual in published research, where constructs are measuring different things.
Question three, examining the relationship among the drivers of use, further employs structural equation modelling. In this section, it becomes clear that the authors have confused their table numbers – they refer to Table 5 for the results of the mediated relationships, but these are actually in Table 7. There is a path diagram representing the relationships between constructs (Figure 3), but these are inconsistent with the reporting in text and tables. For example, the mediation of experience on the relationship between performance expectancy and behavioural intention to use generative AI is negative on the diagram but is described as positive in the text and in Table 8. Similarly, they claim that experience negatively moderates the relationship between social influence and behavioural intention, while the diagram shows a positive moderation. This is important and undermines confidence in the quality of the analysis.
Overall, I’m not impressed with the quality of the results. The research seems to be confused, haphazard and error prone, with poor attention to detail and inconsistent epistemology and ontology. In the Open University framework, this suggests poor performance in the ‘Presentation’ criteria. I’m not convinced that using the UTAUT2 is necessary to understand the drivers of use of generative AI, and the results confuse the use of generative AI by educators and students – talking both about what students might be allowed to use, and how educators might also find uses for it. It seems unlikely that the UTAUT2 is appropriate for both purposes. Therefore, I think this also does poorly on the ‘Relevance’ criteria.
Having considered the results and quality of the analysis, we now move on to considering how the authors have interpreted their findings within the discussion. The discussion starts with a generic description that does not reflect the findings. In fact, there seems to be little real depth of discussion regarding the findings of the study at all! The discussion is divided into sections, which have no clear relationship with the research questions that were set out. The comments are overly general regarding the use of AI for assessing students. Furthermore, I was surprised to see that in the theoretical implications, a different theoretical model (UTUAT) to the one listed in the methodology.
In the discussion section, qualitative findings that weren’t reported in the results were reported, for example an overall concern regarding academic integrity. The ‘moderating role of experience’ made claims that experienced educators were likely to see the benefits of generative AI, but this isn’t supported by any of the evidence presented. When considering the implications of the research, the authors uncritically imply that adoption of generative AI is desirable in education. This bias suggests the paper also doesn’t do well in the PROMPT framework for objectivity. From section 6.4.2 in the paper, the format is surprisingly similar to that which is often generated by generative AI itself, calling into question the authorship of this work. It is vague, and doesn’t reflect the specific findings of the paper, or indeed any references to other work.
Poor Publishers & Slop
In the Open University framework, the issue of provenance is mentioned regarding the publication methods, the journal and the peer review process. Within the context of this paper, there are some questions to be addressed regarding the publisher. MDPI is a poor-quality publisher with a history of publishing poor science with little or no peer review (Beall, 2015). Indeed, the publicly available peer reviews of the paper are very weak (https://www.mdpi.com/2227-7102/14/10/1090/review_report). MDPI has been implicated in paper-mill scandals and accused of publishing pseudoscience and has a reputation of self-citing its own journals. These criticisms have led to the publisher being having journals delisted from Web of Science (Brainard, 2023). Publishing in this journal is attractive due to the quick turnaround of papers. Although the peer-review process has faced criticism, this speed is beneficial for GenAI research, which is a rapidly evolving field. The urgency of sharing and discussing new findings may outweigh concerns about the publisher’s reputation. However, this does not negate the reputation of this publisher, or the negative claims made against it. There are better solutions for quick publishing, such as one of the many open science pre-print websites where papers can be published, together with access to the data for other researchers to interrogate and discuss. This would give more authenticity to the research and would allow for the paper to be published later through a more reputable publisher after peer review.
I suspect that some of this paper has been AI-generated. I have been reading and writing about generative AI for over two years and have quickly become accustomed to the type of content that it produces. Whilst on one hand generative AI can be used as a tool to aid the development of research, creating writing frames, or for development of ideas, the generation of content through generative AI is problematic for the academic community. It becomes part of the noise, or what has been described as AI slop. The production of more and more papers in this way is a function of the crisis in worldwide academia, and the pressure to create papers is the latest iteration of the academic paper mill. If GPT-created papers proliferate, this impacts the whole community. As Hern & Milmo (2024) point out, wading through this content will have a negative effect on knowledge due to the time and effort taken. Copstake et al. (2024) describe generated text within research papers as having little or no value, and that large language models such as ChatGPT are becoming “supersloppers”, generating more and more content with little value or even accuracy. The inevitable outcome for this is as Henderson (2024) state “academic writing will be increasingly offloaded to AI. Just type in a prompt, upload your data sheets, and tell the AI how comfortable you are with p-hacking, and then you’ll have a full academic article worthy of a psychology journal…” This development does not bode well for the rigour and validity of academic publishing for the future.
New frameworks for the age of AI
There have been notorious examples of papers that have used large language models to illustrate the ability for generative AI to create convincing content (Cotton & Cotton, 2023). However, this emphasises the need to develop new frameworks that can be used to assess the quality, validity and usefulness of research on use of generative AI in education. Existing frameworks may be useful for analysing the research approach and methodology of papers, but they do not address the issue of generated content. Whilst well-edited, reasoned argument may be of some value, we need to develop ways of evaluating content that may have been AI-created.
One of the questions that I ask myself when looking at a paper concern consistency. For example, in this paper, the methodology is not consistent about which framework was used. UTAUT is mentioned, as is UTAUT2. These are different frameworks – UTAUT2 being a later development of UTAUT which is itself a development of TAM. We could be generous and put this down to bad copy editing, but the precision here about which model is being employed is important, as without being certain, we cannot cross-reference the model. Issues around the text being very generalised and not specific enough to the research question could be another indicator of poor-quality AI generation, as is the very surface approach to thematic analysis and Braun & Clark’s work. Ironically, this paper itself calls for robust frameworks to protect academic integrity when using AI.
Therefore, a new framework for assessing academic papers could focus on several key indicators of potential AI generation. These include consistency in theoretical frameworks and methodology, formulaic language patterns, generic or superficial examples, inconsistency in terminology usage, and a lack of meaningful engagement with cited papers.
Finally
In undertaking this assignment, I have learnt many things. The analysis of the paper itself has not been the only learning outcome. I have re-engaged with philosophy textbooks from when I was first at university over 30 years ago and re-read Flew which has been enjoyable. A level maths was a really long time ago, and I really need to study some statistics before writing my dissertation! My choice of paper has led to investigating many other interesting areas. I hadn’t been aware of the academic publishing scandals, and the differences in working practices of publishers. As ever, it’s a case of follow the money, private profit over public good. In the area of AI, the concept of slop was new, and this has resonated with me. If research is generated, churned out in questionable journals, and then used uncritically by policy makers in education then the technology will be doing more harm than good. This heightens the importance of critical evaluation and the need of evaluative frameworks in the age of AI.
References
Beall, J. (2015, 17 December). Instead of a Peer Review, Reviewer Sends Warning to Authors. Scholarly Open Access. https://web.archive.org/web/20160313073101/https://scholarlyoa.com/2015/12/17/instead-of-a-peer-review-reviewer-sends-warning-to-authors/
Biesta, G. (2020). Educational Research: An unorthodox introduction. Bloomsbury https://www.bloomsbury.com/uk/educational-research-9781350097988/
Birtill, M., & Birtill, P. (2024). Implementation and Evaluation of GenAI-Aided Tools in a UK Further Education College. In Artificial Intelligence Applications in Higher Education (pp. 195-214). Routledge. https://www.taylorfrancis.com/chapters/edit/10.4324/9781003440178-12/implementation-evaluation-genai-aided-tools-uk-education-college-mike-birtill-pam-birtill
Brainard, J. (2023, March 28). Fast-growing open-access journals stripped of coveted impact factors. Science. https://www.science.org/content/article/fast-growing-open-access-journals-stripped-coveted-impact-factors
Braun, V., & Clarke, V. (2022). Thematic analysis: A practical guide. SAGE. https://uk.sagepub.com/en-gb/eur/thematic-analysis/book248481
Braun, V., & Clarke, V. (2024). Reporting guidelines for qualitative research: a values-based approach. Qualitative Research in Psychology, 1–40. https://doi.org/10.1080/14780887.2024.2382244
Buckler, S., & Moore, H. (2023). Essentials of Research Methods in Education. SAGE. https://uk.sagepub.com/en-gb/eur/essentials-of-research-methods-in-education/book279681
CASP. (n.d.). Critical Appraisal Checklists. Retrieved December 19, 2024, from https://casp-uk.net/casp-tools-checklists/
Coe, R. (2021). The Nature of Educational Research. In Coe, R., Waring, M., Hedges, L., & Day Ashley, L. (Eds.) (2021). Research Methods and Methodologies in Education. (3 ed.) SAGE Publications. https://us.sagepub.com/en-us/nam/research-methods-and-methodologies-in-education/book271175#description
Cotton, D. R. E., Cotton, P. A., & Shipway, J. R. (2023). Chatting and cheating: Ensuring academic integrity in the era of ChatGPT. Innovations in Education and Teaching International, 61(2), 228–239. https://doi.org/10.1080/14703297.2023.2190148
Copestake, A., Duggan, L., Herbelot, A., Moeding, A., & von Redecker, E. (2024). LLMs as supersloppers. Cambridge Open Engage. https://doi.org/10.33774/coe-2024-dx12p
Flew, A. (1989). An introduction to western philosophy: Ideas and argument from Plato to Popper. Thames and Hudson.
Greenhalgh, T. (2019). How to Read a Paper (6th ed.). Wiley-Blackwell https://www.wiley.com/en-ae/How+to+Read+a+Paper%3A+The+Basics+of+Evidence-based+Medicine+and+Healthcare%2C+6th+Edition-p-9781119484745
Henderson, J. (2024, September 11). Academic publishing sells out to AI. Commonplace Philosophy. https://jaredhenderson.substack.com/p/academic-publishing-sells-out-to
Hern, A., & Milmo, D. (2024, May 19). Spam, junk … slop? The latest wave of AI behind the ‘zombie internet’. The Guardian. https://www.theguardian.com/technology/article/2024/may/19/spam-junk-slop-the-latest-wave-of-ai-behind-the-zombie-internet
In’nami, Y., Koizumi, R. (2013). Structural Equation Modeling in Educational Research. In: Khine, M.S. (eds) Application of Structural Equation Modeling in Educational Research and Practice. Contemporary Approaches to Research in Learning Innovations. SensePublishers, Rotterdam. https://doi.org/10.1007/978-94-6209-332-4_2
Kang, H., & Ahn, J. W. (2021). Model setting and interpretation of results in research using structural equation modeling: A checklist with guiding questions for reporting. Asian Nursing Research, 15(3), 157-162. https://www.asian-nursingresearch.com/article/S1976-1317(21)00042-6/fulltext
Khlaif, Z. N., Ayyoub, A., Hamamra, B., Bensalem, E., Mitwally, M. A. A., Ayyoub, A., Hattab, M. K., & Shadid, F. (2024). University Teachers’ Views on the Adoption and Integration of Generative AI Tools for Student Assessment in Higher Education. Education Sciences, 14(10), 1090. https://doi.org/10.3390/educsci14101090
Kwon, D. (2024, December 09). Publishers are selling papers to train AIs — and making millions of dollars. Nature 636, 529-530. https://doi.org/10.1038/d41586-024-04018-5
Robson, C. (2014) Real World Research 3rd edition. Wiley https://www.wiley.com/en-gb/Real+World+Research%2C+3rd+Edition-p-9781119959205
Tamilmani, K., Rana, N. P., Wamba, S. F., & Dwivedi, R. (2021). The extended Unified Theory of Acceptance and Use of Technology (UTAUT2): A systematic literature review and theory evaluation. International Journal of Information Management, 57, 102269. https://doi.org/10.1016/j.ijinfomgt.2020.102269
The Open University. (n.d.). Evaluation using PROMPT. Retrieved December 19, 2024, from https://www5.open.ac.uk/library/help-and-support/advanced-evaluation-using-prompt
The Russell Group. (2023). Russell Group principles on the use of generative AI tools in education. https://russellgroup.ac.uk/media/6137/rg_ai_principles-final.pdf
Venkatesh, V., Thong, J. Y., & Xu, X. (2012). Consumer acceptance and use of information technology: extending the unified theory of acceptance and use of technology. MIS quarterly, 157-178. https://ssrn.com/abstract=2002388