Author: Mike Birtill
From Paradigms to Practice: Navigating Educational Research in the AI Era
Introduction
It is vital that education uses research in order to make evidence-informed decisions. Therefore, educators need to be able to understand and evaluate published research.
In this blog post I’m going to discuss research used in education. I will give an overview quantitative, qualitative and mixed-methods research. I will then evaluate a paper in my research area – educators’ use of generative AI.
Positions & Paradigms
In education research, it is important to consider the philosophical approach of the researcher to the research being undertaken. This can give us an understanding of the ontological and epistemological assumptions of the researcher, and should inform their approach to designing, conducting and analysing their research. Ontology is the philosophical concept of the study of being. The word comes from Greek, “ontos” – being, and “logia” – which is logical discourse. Antony Flew (1989) describes ontology as being “concerned with what there is.” In the context of education research Buckler & Moore (2023, p35) state that ontology is “a quest for understanding whether to work alone or to work with others.” That is, whether the nature of reality is objective and independent (a realist ontology) or socially constructed (relativist ontology). Epistemology, again from the Greek meaning the logical discourse of knowledge, asks the question “what is knowledge?” (Plato). It is a way of thinking about how we acquire knowledge and whether there are any limits to what can be known. In educational research we use epistemology to frame our thinking around the questions of how our knowledge is constructed. Braun & Clarke (2022) discuss epistemology as a method of evaluating the credibility, authenticity, and reliability of information. Axiology is the study of value – axia is Greek for value or worth. In philosophy axiology explores the values of ethics and aesthetics, investigating the nature of value and value judgements, essentially interrogating what is considered “good”. Within education research we use axiology to assess the value judgements or the value position of the research in order to place it in context. Buckler and Moore (2023) state that axiology “relates to what is ethical and unethical.”
There are several philosophical positions within research that we must consider: positivism, post-positivism, realism, interpretivism, and pragmatism. These define the research methodology and position it within a philosophical framework. Post-positivists view the world from a critical realist perspective. They believe that while there is an objective reality, our understanding of it is problematic and imperfect. Conversely, positivists see the world as an absolute reality – what can be observed is the ultimate reality, accessible through the senses. Within research, positivists would argue that the only legitimate knowledge is that which is gained through the scientific method. Realists believe that knowledge can exist independently of the knowledge held by an individual. It is a form of objectivism, which is the belief that reality exists independently of human perception or understanding. Interpretivists, on the other hand, focus on the subjective nature of reality, emphasising that knowledge and understanding come from interpreting human experience. They argue that reality is socially constructed and shaped by cultural, historical, and contextual factors. Interpretivism often relies on qualitative methodologies to explore the meaning, context, and perspectives of individuals. Pragmatism, meanwhile, takes a more flexible stance, asserting that the value of knowledge lies in its practical application and usefulness. This philosophical approach prioritises solving problems and addressing real-world concerns, blending methodologies as needed to achieve the research objectives. Pragmatism recognises that both objective and subjective perspectives can contribute meaningfully to knowledge creation. It is a rejection of the need place research and be constrained by a notional philosophical paradigm. Coe (2021) defines pragmatism as an anti-philosophical stance while Biesta (2020) regards pragmatism a rejection of paradigms altogether, seeing them as problematic.
It is important to consider these philosophical positions when reading research, as the position taken by a researcher helps to understand what perspective or influence that researcher has placed on their research question, data collection methods, and conclusions in the study. The philosophical positions inform the choice of methods used in research, and the methodological approach. Generally, there are qualitative and quantitative forms of research. However, some studies take a mixed-methods approach combining elements from both. Qualitative research tries to understand experiences through the perspective of the individual. Researchers using this method often collect data by asking questions of the participants and then develop themes from the resulting data. Braun & Clarke (2022) describe qualitative research as being focused on meaning and that it contributes to the “rich tapestry of understanding.” Quantitative research, however, is often based around the idea that there is a singular measurable truth – a realist ontology. In this type of research, the research is claimed to be objective and impartial, with no possibility of researcher bias impacting the data. Data collected in this way is numerical and usually subject to statistical analysis to test specific hypotheses. Robson (2014) states that quantitative research follows a scientific approach to research using the same principles as research undertaken in the natural sciences.
Checklists & Questions
To examine the usefulness of any given paper, it needs to undergo a critical appraisal. Greenhalgh (2019) suggests some questions that we should ask about a paper, namely:
- “Why was the study needed, and what was the research question?”
- “What was the research design?”
- “Was the research design appropriate to the question?”
We can use these questions to initially address a paper, considering the fundamental issues of the research, and whether the research has value. Once we have ascertained the relevance and value of the study, we can then move on to a more critical analysis. In the context of education research, there are several evaluative frameworks that can be employed. The Open University suggests the PROMPT framework (see table below) as a way of performing a critical analysis of literature.
Presentation | considers the clarity and professionalism |
Relevance | ensures the source aligns with the research question |
Objectivity | assesses the neutrality and bias within the paper |
Method | examines how the information was gathered and presented |
Provenance | investigating the credibility and expertise of the author or publisher |
Timeliness | checks how current and appropriate the source is |
The PROMPT framework. https://www5.open.ac.uk/library/help-and-support/advanced-evaluation-using-prompt
By using these criteria, the Open University suggests that we can make a reliable assessment of the quality, validity and usefulness of any given study.
Another source of frameworks to consider is CASP – the Critical Appraisal Skills Programme – as they provide downloadable checklists with prompts to critically evaluate various types of research. The checklists aim to assess the validity of the research by assessing the methodological rigour through appropriateness of design and the minimisation of bias. They check the results and findings to ensure the reliability of the results and if they are credible and measured appropriately. Finally, the checklists seek to evaluate the relevance of the study to local contexts, practitioners and policymakers. CASP aims to provide an accessible, standardised and consistent way of assessing research whilst being evidence-based and easy to use. Other frameworks can be found by searching literature, when necessary, to support evaluation of specific methodological approaches.
There is clearly some overlap between these methods; however, when used in conjunction with each other, we can assess a paper and identify areas where one method might not be as strong, allowing us to gain a broader insight into the research presented. Each framework has its own strengths: for example, Greenhalgh’s questions provide an initial assessment framework, whilst PROMPT offers a comprehensive evaluative structure to guide our analysis. Similarly, CASP is methodological and prompts the reviewer with questions to ensure a consistent analysis has been conducted. By combining these approaches, we can thoroughly evaluate the research in terms of rigour, validity, relevance, and implications for future research.
The Paper:
The paper I have chosen to analyse is Khlaif et al., (2024), “University teachers’ views on the adoption and integration of generative AI tools for student assessment in higher education.” This was published in Education Sciences, in October 2024. I have chosen this paper as it is similar to research I am considering for my own dissertation, being concerned with educator attitudes to AI use and whether the potential benefits are realised. The combination of its contemporary relevance, use of a mixed-methods methodology, and discussion of technology acceptance frameworks, which I also plan to employ in my own research, make this paper particularly interesting for analysis. A copy of the paper can be found here: https://doi.org/10.3390/educsci14101090.
Taking Greenhalgh’s (2019) questions first, I will now describe and then evaluate the paper.
“Why was the study needed, and what was the research question?”
The study is needed to improve understanding of the use of generative AI, especially around student assessment. Generative AI, which has been developing rapidly since early 2022, creates text in response to prompts. It has been seen as a threat to academic integrity, but also potentially useful to alleviate workload issues in education (Birtill & Birtill, 2024). There were three research questions, centred around the issue of how academics use generative AI to assess students, and understanding the factors that drive academics to use generative AI. The final sentence of the introduction states that the research aims to understand the decisions of instructors and professors who doubt the validity of generative AI in student assessment, in order to persuade them otherwise.
“What was the research design?”
The research adopts a mixed-methods approach, with a cross-sectional questionnaire including both open-ended and Likert-scale questions. The participants were self-identified generative AI early-adopter academics in Middle Eastern Universities. Structural equation modelling was used to analyse the quantitative aspects, and a thematic analysis was employed to understand the qualitative responses. The researchers used the extended unified theory of acceptance and use of technology (UTAUT2, Venkatesh 2012) to frame their analysis. This model uses concepts such as expectancy of technology, habit of use, and behavioural intentions, to predict actual use of a technology. This indicate that the research is aligned with a positivist approach, using a theoretical framework, and statistical analysis with a large sample size.
“Was the research design appropriate to the question?”
The research design was partially appropriate. To understand educators’ attitudes towards a new technology, it is sensible to employ an existing, theory-informed framework such as UTAUT2. The framework uses Likert-scale questions, which have been validated in a several different contexts. Indeed, the UTAUT2 has over 6,000 citations (Tamilmani et al., 2021). However, such a quantitative approach does suggest a realist ontology. This seems at odds with a research question that is investigating a socially constructed attitude. However, use of a qualitative component to supplement the quantitative analysis does at least partially address this. The researchers are not explicit about their ontological or epistemological positions, making it difficult to evaluate the appropriateness of the design and methodology they have adopted. However, the research is motivated by the need to persuade reluctant academics to use generative AI and so examining motivations in a group of early adopters seems inappropriate. More problematic, there is no discussion of ethics in the paper. It isn’t clear how consent was obtained from participants, and whether there was any ethical review of the research.
Having gained our bearings, and described the approach of the paper, we can now employ more detailed frameworks to continue our evaluation. Let’s first consider each research question in turn. The first question examines how academics use generative AI to assess their students and is addressed by a qualitative analysis of the open text answers. The CASP checklist for qualitative studies (CASP, n.d.) asks whether the results are valid, and what the results are, and if the results will help locally. Another approach to assessing qualitative analysis is the Braun and Clarke ‘Bingo’ card (see below). More seriously, Braun and Clarke have recently published their own reporting guidelines for qualitative research (Braun and Clarke, 2024). They discuss ‘big Q’ and ‘small q’ qualitative research. The results reported in Khalif et al., (2024) are certainly ‘small q’. There is no discussion of deeper meanings, or the contextual nature of the open text responses.
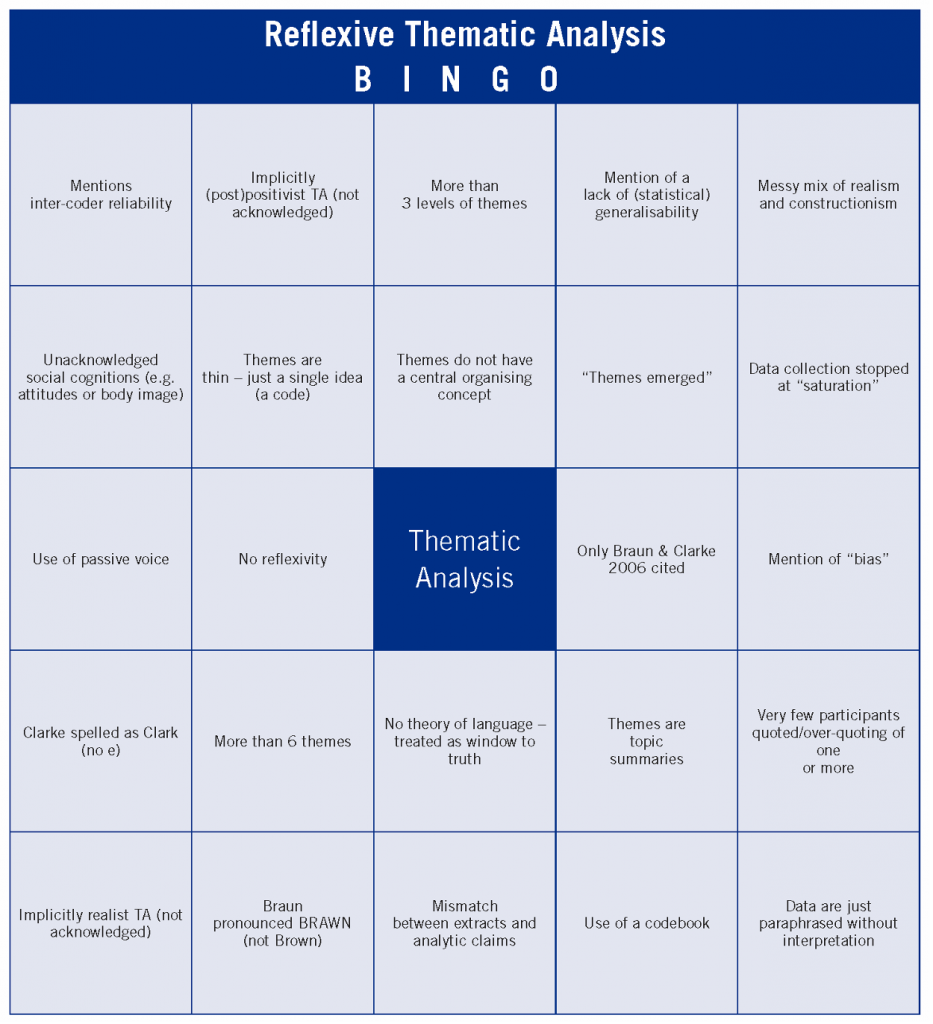
The paper claims to have used an inductive thematic analysis. However, an inductive thematic analysis would be used to develop ideas found in the data, finding patterns and themes in the data. However, the researchers here started with a theoretical framework of UTAUT2 and are using this model to test their thesis. In essence, they have conducted a deductive analysis where they want to confirm their hypothesis and have coded the responses to match the theoretical model. The paper suffers from what Braun & Clarke describe as positivism creep – the researchers are clearly valuing objectivity in the study and looking for an ultimate truth. The authors do not give any detailed description of their thematic coding, not even listing the six-stage process of Braun and Clarke. In their results, they report two themes, but these are closer to ‘topic summaries’ (Braun and Clarke, 2022) which describe the content of answers rather than the underlying themes, with data paraphrased or even quoted without interpretation. In addition, researchers using thematic analysis should state their positionality regarding their research position and consider how their paradigm influences the interpretation of the data. In this case, that has not been included.
From the qualitative analysis, the authors created a helpful flowchart showing the process that academics use to develop AI supported assessments. However, in the text, they seem to infer that this is a model that should be encouraged, rather than reflecting the process described by their participants: “The flowchart highlights the iterative nature of this process, encouraging educators to continually refine their methods” (p1090). This is confusing, and it isn’t clear how this flowchart relates to the qualitative data.
The second part of the qualitative analysis summarises three approaches to using generative AI in assessment. These align with other approaches that have been developed institutionally, for example The Russell Group guidelines (Russell Group, 2023) although the authors do not explicitly draw out these similarities. These approaches are banning generative AI, using it in a supportive capacity, and encouraging full use of generative AI in assessment. In this section, there is again little depth of analysis, and quotes are given with no interpretation.
The second research question, examining the factors that drive academics to use generative AI in assessment, is addressed by both qualitative and quantitative approaches. However, the qualitative section includes no quotes, and only a list of relevant factors.
The quantitative approach is to examine how well the UTAUT2 explains participants use of generative AI, using structural equation modelling (SEM). This is a statistical technique that allows the researcher to model the relationships between several constructs, and to understand which constructs predict each other (In’nami & Koizumi, 2013). Constructs are comprised of responses to items on the Likert questionnaire. I am not an expert in statistical analysis and feel unable to fully evaluate the claims made here. However, from looking at the data presented there are clear inconsistencies in the reporting and presentation of the results. This indicates a lack of precision and makes me question the validity of their claims.
The authors present the results of the SEM, but they don’t present any of the descriptive statistics. The ‘outcome’ measure they are trying to predict is ‘Use of generative AI’ on a 1-7 scale – but we are not told what the average use, or range of scores of their participants are. Given the inherent bias of including explicitly early adopters of AI in their sample this seems like a real omission.
In Table 5, the authors present the results of the SEM. P-values are provided, stating p=0.00. However, this isn’t appropriate, and p is never exactly equal to zero. All their hypotheses are supported in these statistics, with positive relationships between most of the constructs. These strong positive correlations between the constructs are also in table 4 – although this is only clear from reading the text, as the table caption is incomplete! As a non-expert, it seems slightly troubling that everything correlates so highly with each other. This is unusual in published research, where constructs are measuring different things.
Question three, examining the relationship among the drivers of use, further employs structural equation modelling. In this section, it becomes clear that the authors have confused their table numbers – they refer to Table 5 for the results of the mediated relationships, but these are actually in Table 7. There is a path diagram representing the relationships between constructs (Figure 3), but these are inconsistent with the reporting in text and tables. For example, the mediation of experience on the relationship between performance expectancy and behavioural intention to use generative AI is negative on the diagram but is described as positive in the text and in Table 8. Similarly, they claim that experience negatively moderates the relationship between social influence and behavioural intention, while the diagram shows a positive moderation. This is important and undermines confidence in the quality of the analysis.
Overall, I’m not impressed with the quality of the results. The research seems to be confused, haphazard and error prone, with poor attention to detail and inconsistent epistemology and ontology. In the Open University framework, this suggests poor performance in the ‘Presentation’ criteria. I’m not convinced that using the UTAUT2 is necessary to understand the drivers of use of generative AI, and the results confuse the use of generative AI by educators and students – talking both about what students might be allowed to use, and how educators might also find uses for it. It seems unlikely that the UTAUT2 is appropriate for both purposes. Therefore, I think this also does poorly on the ‘Relevance’ criteria.
Having considered the results and quality of the analysis, we now move on to considering how the authors have interpreted their findings within the discussion. The discussion starts with a generic description that does not reflect the findings. In fact, there seems to be little real depth of discussion regarding the findings of the study at all! The discussion is divided into sections, which have no clear relationship with the research questions that were set out. The comments are overly general regarding the use of AI for assessing students. Furthermore, I was surprised to see that in the theoretical implications, a different theoretical model (UTUAT) to the one listed in the methodology.
In the discussion section, qualitative findings that weren’t reported in the results were reported, for example an overall concern regarding academic integrity. The ‘moderating role of experience’ made claims that experienced educators were likely to see the benefits of generative AI, but this isn’t supported by any of the evidence presented. When considering the implications of the research, the authors uncritically imply that adoption of generative AI is desirable in education. This bias suggests the paper also doesn’t do well in the PROMPT framework for objectivity. From section 6.4.2 in the paper, the format is surprisingly similar to that which is often generated by generative AI itself, calling into question the authorship of this work. It is vague, and doesn’t reflect the specific findings of the paper, or indeed any references to other work.
Poor Publishers & Slop
In the Open University framework, the issue of provenance is mentioned regarding the publication methods, the journal and the peer review process. Within the context of this paper, there are some questions to be addressed regarding the publisher. MDPI is a poor-quality publisher with a history of publishing poor science with little or no peer review (Beall, 2015). Indeed, the publicly available peer reviews of the paper are very weak (https://www.mdpi.com/2227-7102/14/10/1090/review_report). MDPI has been implicated in paper-mill scandals and accused of publishing pseudoscience and has a reputation of self-citing its own journals. These criticisms have led to the publisher being having journals delisted from Web of Science (Brainard, 2023). Publishing in this journal is attractive due to the quick turnaround of papers. Although the peer-review process has faced criticism, this speed is beneficial for GenAI research, which is a rapidly evolving field. The urgency of sharing and discussing new findings may outweigh concerns about the publisher’s reputation. However, this does not negate the reputation of this publisher, or the negative claims made against it. There are better solutions for quick publishing, such as one of the many open science pre-print websites where papers can be published, together with access to the data for other researchers to interrogate and discuss. This would give more authenticity to the research and would allow for the paper to be published later through a more reputable publisher after peer review.
I suspect that some of this paper has been AI-generated. I have been reading and writing about generative AI for over two years and have quickly become accustomed to the type of content that it produces. Whilst on one hand generative AI can be used as a tool to aid the development of research, creating writing frames, or for development of ideas, the generation of content through generative AI is problematic for the academic community. It becomes part of the noise, or what has been described as AI slop. The production of more and more papers in this way is a function of the crisis in worldwide academia, and the pressure to create papers is the latest iteration of the academic paper mill. If GPT-created papers proliferate, this impacts the whole community. As Hern & Milmo (2024) point out, wading through this content will have a negative effect on knowledge due to the time and effort taken. Copstake et al. (2024) describe generated text within research papers as having little or no value, and that large language models such as ChatGPT are becoming “supersloppers”, generating more and more content with little value or even accuracy. The inevitable outcome for this is as Henderson (2024) state “academic writing will be increasingly offloaded to AI. Just type in a prompt, upload your data sheets, and tell the AI how comfortable you are with p-hacking, and then you’ll have a full academic article worthy of a psychology journal…” This development does not bode well for the rigour and validity of academic publishing for the future.
New frameworks for the age of AI
There have been notorious examples of papers that have used large language models to illustrate the ability for generative AI to create convincing content (Cotton & Cotton, 2023). However, this emphasises the need to develop new frameworks that can be used to assess the quality, validity and usefulness of research on use of generative AI in education. Existing frameworks may be useful for analysing the research approach and methodology of papers, but they do not address the issue of generated content. Whilst well-edited, reasoned argument may be of some value, we need to develop ways of evaluating content that may have been AI-created.
One of the questions that I ask myself when looking at a paper concern consistency. For example, in this paper, the methodology is not consistent about which framework was used. UTAUT is mentioned, as is UTAUT2. These are different frameworks – UTAUT2 being a later development of UTAUT which is itself a development of TAM. We could be generous and put this down to bad copy editing, but the precision here about which model is being employed is important, as without being certain, we cannot cross-reference the model. Issues around the text being very generalised and not specific enough to the research question could be another indicator of poor-quality AI generation, as is the very surface approach to thematic analysis and Braun & Clark’s work. Ironically, this paper itself calls for robust frameworks to protect academic integrity when using AI.
Therefore, a new framework for assessing academic papers could focus on several key indicators of potential AI generation. These include consistency in theoretical frameworks and methodology, formulaic language patterns, generic or superficial examples, inconsistency in terminology usage, and a lack of meaningful engagement with cited papers.
Finally
In undertaking this assignment, I have learnt many things. The analysis of the paper itself has not been the only learning outcome. I have re-engaged with philosophy textbooks from when I was first at university over 30 years ago and re-read Flew which has been enjoyable. A level maths was a really long time ago, and I really need to study some statistics before writing my dissertation! My choice of paper has led to investigating many other interesting areas. I hadn’t been aware of the academic publishing scandals, and the differences in working practices of publishers. As ever, it’s a case of follow the money, private profit over public good. In the area of AI, the concept of slop was new, and this has resonated with me. If research is generated, churned out in questionable journals, and then used uncritically by policy makers in education then the technology will be doing more harm than good. This heightens the importance of critical evaluation and the need of evaluative frameworks in the age of AI.
References
Beall, J. (2015, 17 December). Instead of a Peer Review, Reviewer Sends Warning to Authors. Scholarly Open Access. https://web.archive.org/web/20160313073101/https://scholarlyoa.com/2015/12/17/instead-of-a-peer-review-reviewer-sends-warning-to-authors/
Biesta, G. (2020). Educational Research: An unorthodox introduction. Bloomsbury https://www.bloomsbury.com/uk/educational-research-9781350097988/
Birtill, M., & Birtill, P. (2024). Implementation and Evaluation of GenAI-Aided Tools in a UK Further Education College. In Artificial Intelligence Applications in Higher Education (pp. 195-214). Routledge. https://www.taylorfrancis.com/chapters/edit/10.4324/9781003440178-12/implementation-evaluation-genai-aided-tools-uk-education-college-mike-birtill-pam-birtill
Brainard, J. (2023, March 28). Fast-growing open-access journals stripped of coveted impact factors. Science. https://www.science.org/content/article/fast-growing-open-access-journals-stripped-coveted-impact-factors
Braun, V., & Clarke, V. (2022). Thematic analysis: A practical guide. SAGE. https://uk.sagepub.com/en-gb/eur/thematic-analysis/book248481
Braun, V., & Clarke, V. (2024). Reporting guidelines for qualitative research: a values-based approach. Qualitative Research in Psychology, 1–40. https://doi.org/10.1080/14780887.2024.2382244
Buckler, S., & Moore, H. (2023). Essentials of Research Methods in Education. SAGE. https://uk.sagepub.com/en-gb/eur/essentials-of-research-methods-in-education/book279681
CASP. (n.d.). Critical Appraisal Checklists. Retrieved December 19, 2024, from https://casp-uk.net/casp-tools-checklists/
Coe, R. (2021). The Nature of Educational Research. In Coe, R., Waring, M., Hedges, L., & Day Ashley, L. (Eds.) (2021). Research Methods and Methodologies in Education. (3 ed.) SAGE Publications. https://us.sagepub.com/en-us/nam/research-methods-and-methodologies-in-education/book271175#description
Cotton, D. R. E., Cotton, P. A., & Shipway, J. R. (2023). Chatting and cheating: Ensuring academic integrity in the era of ChatGPT. Innovations in Education and Teaching International, 61(2), 228–239. https://doi.org/10.1080/14703297.2023.2190148
Copestake, A., Duggan, L., Herbelot, A., Moeding, A., & von Redecker, E. (2024). LLMs as supersloppers. Cambridge Open Engage. https://doi.org/10.33774/coe-2024-dx12p
Flew, A. (1989). An introduction to western philosophy: Ideas and argument from Plato to Popper. Thames and Hudson.
Greenhalgh, T. (2019). How to Read a Paper (6th ed.). Wiley-Blackwell https://www.wiley.com/en-ae/How+to+Read+a+Paper%3A+The+Basics+of+Evidence-based+Medicine+and+Healthcare%2C+6th+Edition-p-9781119484745
Henderson, J. (2024, September 11). Academic publishing sells out to AI. Commonplace Philosophy. https://jaredhenderson.substack.com/p/academic-publishing-sells-out-to
Hern, A., & Milmo, D. (2024, May 19). Spam, junk … slop? The latest wave of AI behind the ‘zombie internet’. The Guardian. https://www.theguardian.com/technology/article/2024/may/19/spam-junk-slop-the-latest-wave-of-ai-behind-the-zombie-internet
In’nami, Y., Koizumi, R. (2013). Structural Equation Modeling in Educational Research. In: Khine, M.S. (eds) Application of Structural Equation Modeling in Educational Research and Practice. Contemporary Approaches to Research in Learning Innovations. SensePublishers, Rotterdam. https://doi.org/10.1007/978-94-6209-332-4_2
Kang, H., & Ahn, J. W. (2021). Model setting and interpretation of results in research using structural equation modeling: A checklist with guiding questions for reporting. Asian Nursing Research, 15(3), 157-162. https://www.asian-nursingresearch.com/article/S1976-1317(21)00042-6/fulltext
Khlaif, Z. N., Ayyoub, A., Hamamra, B., Bensalem, E., Mitwally, M. A. A., Ayyoub, A., Hattab, M. K., & Shadid, F. (2024). University Teachers’ Views on the Adoption and Integration of Generative AI Tools for Student Assessment in Higher Education. Education Sciences, 14(10), 1090. https://doi.org/10.3390/educsci14101090
Kwon, D. (2024, December 09). Publishers are selling papers to train AIs — and making millions of dollars. Nature 636, 529-530. https://doi.org/10.1038/d41586-024-04018-5
Robson, C. (2014) Real World Research 3rd edition. Wiley https://www.wiley.com/en-gb/Real+World+Research%2C+3rd+Edition-p-9781119959205
Tamilmani, K., Rana, N. P., Wamba, S. F., & Dwivedi, R. (2021). The extended Unified Theory of Acceptance and Use of Technology (UTAUT2): A systematic literature review and theory evaluation. International Journal of Information Management, 57, 102269. https://doi.org/10.1016/j.ijinfomgt.2020.102269
The Open University. (n.d.). Evaluation using PROMPT. Retrieved December 19, 2024, from https://www5.open.ac.uk/library/help-and-support/advanced-evaluation-using-prompt
The Russell Group. (2023). Russell Group principles on the use of generative AI tools in education. https://russellgroup.ac.uk/media/6137/rg_ai_principles-final.pdf
Venkatesh, V., Thong, J. Y., & Xu, X. (2012). Consumer acceptance and use of information technology: extending the unified theory of acceptance and use of technology. MIS quarterly, 157-178. https://ssrn.com/abstract=2002388
AI, Education & the Global South
In this case study I will discuss the potential for artificial intelligence (AI) to transform education in the Global South, with a particular focus on the continent of Africa. I will start with a description of AI, and the most recent developments in this field. I will then consider the ways in which AI can be used in education. I will consider some of the ethical issues of AI, before conducting a detailed analysis of Global South perspectives, examining the potential for generative AI to meet the needs of UNESCO Sustainable Development Goal 4, and the potential barriers and enablers to this, with a particular focus on the digital divide.
The use of AI in education was first proposed in 1956 by John McCarthy at Dartford College, USA (Zawacki-Richter et al., 2019). Indeed, Doroudi (2023) argues that AI and education are inextricably linked, as early developments in AI were driven by cognitive scientists. Further, intelligent tutoring systems were posited as an aim of AI as early as 1970. During the 1980s, efforts aimed at developing personalised intelligent tutoring systems evolved into adaptive learning systems in the early 2000s. There are several different technologies that are called artificial intelligence. The distinction between generative AI, as exemplified by tools such as ChatGPT and DALL-E, and machine learning should be made clear. Language based Generative AI (genAI) creates text, based on prompts given by the user. It is based on a large language model (LLM), trained on large sets of text scraped from the internet and other historic sources. This produces impressive content, which then can be further refined by conversation and follow up questions. The text produced by the most recent version of ChatGPT (GPT-4) has been found to be indistinguishable from human written content. It has been claimed that this passes the Turing Test, with the responses to behavioural questions indistinguishable from humans (Mei et al., 2024). In contrast, machine learning, a term invented by Arthur Samuel at IBM in 1959, is based on statistical algorithms that enable computers to learn from and make predictions based on data which has been classified. This enables the computer to learn by identifying patterns and making decisions without explicit program instructions. Some example applications of machine learning are the monitoring of crops to provide better yields and in intelligent management systems (Wall et al., 2021).
Easy access to genAI was provided in November 2022 when OpenAI launched ChatGPT. Since then, there has been a rapid uptake of creation and use of LLMs. This has led to widespread concern about the impact of genAI on education (Memarian & Doleck, 2023), and ensuring the validity of written assessments produced outside of exam conditions (Eke, 2023). There have been claims that LLMs can pass key assessments such as the bar exam (Katz et al., 2024) and medical exams (Ali et al., 2024). This in turn has produced a moral panic in the global north with concerns about the impact on validity of assessment, and potential replacement of teachers by AI tutors (Selwyn, 2019).
There is, however, the potential for education to be positively transformed by this technology. Educational technology companies are investing heavily in AI tools to support teachers in their practice and reduce teacher workload (Slagg, 2023). This is a very seductive offer for not only educators but also for educational establishments and governments who want to be perceived as at the cutting edge of technology. For example, in the UK, the government has invested £2 million in Oak Academy to produce AI tools for schools (Department for Education, 2023) and a further £4 million to reduce workload (Department for Education, 2024). This willingness to use AI is found internationally (Schiff, 2021).
The proposal is that these tools may provide support to educators by creating resources such as lesson plans, and materials for formative assessment of students. However, critics argue that this removes teacher engagement with the material. However, there is evidence that such AI assisted tutoring can improve the teaching of low skilled teachers (Wang et al., 2024). Another use of AI in education is to support students in their own work. Tools such as ChatGPT, it is claimed, can provide students with timely feedback on their work, to support their reflection and self-evaluation (Kestin et al., 2024).
Another long-held ambition for AI in education is the development of adaptive learning technologies (Strielkowski, 2024), which tailor educational content to individual student needs, thereby supporting the diverse needs of learners. Using approaches such as learning analytics, these AI-driven tools, can bridge educational gaps and provide educational resources to underserved communities (Kamalov et al., 2023), whilst enabling teachers to focus on interactive and creative teaching rather than administrative tasks (Pedro et al., 2019). AI is therefore being promoted as a transformational force with the ability to reshape education.
However, the ethics of AI, and the differential impact it may have between the global north and the global south, need to be considered. For example, there is evidence of harm coming to people who have been involved in moderating the training data content for LLMs. Moderators have reported that they have been exposed to racist, violent, and sexist material which has impacted their mental health (Rowe, 2023. Some moderators report that they are suffering from post-traumatic stress having dealt with the content. The role of humans in AI are not exclusive to LLMs. Muldoon et al. (2024) highlight that machine learning involves significant data annotation, classifying data that subsequently drives the learning algorithms. The human processes involved are obscured by the perceived functionality of the AI tool. For example, a typical hour-long video will take over 800 hours of human work to annotate for the AI tool. This low-skilled work is typically done in sub-Saharan Africa, with pay of less than two dollars an hour (Tucker, 2024). This outsourcing to the Global South where labour costs are low perpetuates neo-colonial employment practices.
It can be seen, therefore, that AI in terms of its digital colonialism is an “extraction machine” (Muldoon et al., 2024). They make the analogy that with the introduction of the railways into Africa laid the infrastructure to later extract in the 19th century is the same as the introduction in 2009 of fibre optics in preparation for the digital extraction of the continent. LLMs have been trained, at great cost, by private companies in the global north, and therefore there is a need to find ways of monetising the tools (Merchant, 2024). Thus, they are often marketed, with great hype, as solutions for every educator’s problem. Rather than locally driven solutions and technology in the Global South, this option is replaced with the neo-paternalist extractive solutions designed to maximise profit for the companies of the Global North.
However, some reflexivity is needed here. What I have argued is from my perspective, and I’m WEIRD – a Western, Educated, Industrialised, Rich, and Democratic male from the UK (Linxen et al., 2021). My argument so far could be seen as a form of digital colonialism – one where I project my Western-centric paternalism onto my thinking around the issue. As Arora (2024a) states, my pessimism about AI technology is based upon my position of privilege, and the Global South does not have the luxury of pessimism, only optimism about the possibilities of change that can come through the adoption of generative AI. We, in the Global North, need to hear these voices and include them in our arguments. Arora (2024b) states we need to decolonise our approach to understanding AI in the Global South. We need to ask the questions: what does the Global South want from this technology, why, and what are the factors driving this?
Education is a key component of addressing global inequality (Gethin, 2023). Therefore, any tool that can transform education has the potential to improve global equality. Indeed, UNESCO states that AI has the possibility to address inequality and democratise education (Miao et al., 2021). The UNESCO 17 Sustainable development goals (SDG) concern the economic, social and environmental dimensions of sustainable development. All UN member states have committed themselves to working towards these goals by 2030. SDG 4 concerns education, with the aim to “Ensure inclusive and equitable quality education and promote lifelong learning opportunities for all” (The Global Goals, n.d.). Even before LLMs became prevalent, UNESCO had identified the transformative potential of AI in achieving this goal: “AI holds the potentials to … reducing barriers to access education, automating management processes, analysing learning patterns and optimizing learning processes with a view to improving learning outcomes” (UNESCO, 2019). Additionally, the Africa Union also believes AI has the potential to transform education and help the African Continent to achieve SDG4 (Africa Union, 2024). They wish to realise the potential of AI for development by increasing the digital literacy of teachers, so education can make full use of the potential of AI.
AI’s promise to expand and democratise high-quality education should in turn improve skill development, essential for developing a workforce in rapidly changing job markets. This could therefore contribute to socioeconomic progress and achievement of several Sustainable Development Goals. Already, AI is having an impact on language translation, which can help democratise education and produce inclusive growth across borders (Africa Union, 2024).
The swift rise of generative AI tools and its transformative possibilities in both education and other sectors, has caught the global imagination. The technology has a real “wow factor”. Attractive technology is not a new phenomenon. Novel technological solutions to problems, full of promises, are what Ames (2015) calls “charismatic technology”. The Gartner hype cycle evaluates the performance of technology, separating it from hype to inform commercial investment decisions. Currently, genAI has passed the peak of inflated expectations in this cycle (Gartner, 2024), indicating that there is growing scepticism on the effectiveness of genAI. Therefore, AI can be seen as the latest in a long line of attractive, seductive and charismatic technologies. The principal offer is their promise to solve society’s problems. However, as Ames points out, charismatic machines are ultimately conservative and serve to transmit the status quo or continue a form of technological colonialism (Ames, 2019).
A previous example of a “charisma machine” in education is the One Laptop Per Child project. This project, started by Nicholas Negroponte at MIT, intended to provide ruggedised laptops costing only $100. The laptops were designed to be low-power devices, that used an open-source operating system, with built-in educational software. It was intended that these laptops would be bought by governments and distributed to children throughout the developing world, which would drive discovery learning. Similarly, Sugata Mitra’s Hole in the Wall project provided free access to computers in walls in public spaces. The computers were installed at child height, to be accessible by children and had internet access. It was envisaged that children would self-learn by what Mitra called “minimally invasive education” (Mitra & Rana, 2001). Both utopian ideas assumed that if technology is provided, children will use it to teach themselves anything, from reading to engineering. However, in both cases, this did not happen. Mostly, the machines broke. The infrastructure was not in place to maintain the required internet and computer systems to continue functioning. Hole in the Wall computers were often dominated by older children playing games or viewing inappropriate content (Arora, 2010). In one advertisement for One Laptop Per Child project, the screen was used as a source of illumination at night in a village without electricity. However, the project failed to consider many practicalities, including how the laptop could be recharged without the relevant infrastructure to support it (Ames, 2019). The critical question is whether generative AI will function as a charisma machine, potentially falling short of the high expectations assigned to it.
One way in which AI will fail to meet its potential to improve educational outcomes is if it increases inequality. Giannini (2023), writing for UNESCO, highlights that digital technology has a record of exacerbating inequality. Therefore, if it is to be successful, the implementation of AI in education must be conducted with the aim of closing the digital divide. The digital divide is the notion of the ‘haves’ and ‘have-nots’ with unequal access to digital tools, digital services, education, and the relevant skills needed to navigate a digital landscape. Digital inequality can be categorised through a framework of access. Van Dijk (2020) posits this access is defined as motivational, material, skills, and usage. Motivational access is where there is the motivation to use the technology; material is the possession of computers and internet access, including systems, data and power infrastructure; skills are the actual digital skills that the individual possesses allowing them to use digital devices, also known as digital literacy; and usage is the availability of sufficient time and applications.
This framework can be used to understand the challenges of employing AI in education in the global south, and whether the digital divide is a barrier to effective implementation of AI assisted education. For example, examining previous educational technology solutions through these lenses, it can be seen why initiatives such as One Laptop Per Child ultimately failed. In that case, issues around the robustness of the hardware and internet connectivity stifled the success of the project, with limited access to power and internet connectivity. This is a demonstration of the lack of material access. The domination of the Hole in the Wall computers by older children is example of failure of usage – the target population (younger children) were prevented from having sufficient opportunity to access the computers.
Let us first consider the motivation to use AI in education in the global south. As outlined above, there has been widespread interest in the application of AI in education at a policy level by UNESCO, Africa Union, and other governmental level organisations. Limited research conducted so far demonstrates that there is motivation by educators to use AI (e.g., Khlaif et al., 2024, Kasun et al., 2024). For example, a study examined Nigerian pre-service teachers intentions to use AI in teaching genetics and found that intention to use the tool was predicted by perceived usefulness, aligning with the technology acceptance model (Adelana et al., 2024). For learners, AI can help with structuring essays, improving accessibility or even creating a DALL-E generated interactive learning metaverse (Rospigliosi, 2023). Since the COVID-19 pandemic, which disrupted formal education globally, youth in the global south have been using online tools such as YouTube, TikTok and Instagram to learn skills and develop and share their knowledge, rejecting traditional online learning. This suggests an openness to innovation and ‘global north’ tools (Arora, 2024b). Academic research similarly finds that the human-like qualities of AI chatbots improved Iranian students’ motivation to learn English (Ebadi et al., 2022). Thus, there seems to be widespread motivation to use AI tools in education in the global south.
Next, consider the material access. As previously described, material access includes the physical devices that individuals interact with, as well as the physical infrastructure allowing connection to both power sources and data, including the internet. There have been dramatic improvements recently in data connectivity, with Facebook investing in subsea cables to connect 16 African countries to the internet (Ahmad & Salvadori, 2022), and proving 4G, 5G and broadband access to millions of people on the African continent. This will increase both the capacity and the reliability of internet connections. In contrast to the failure of the One Laptop per Child project, this development makes the implementation of AI within the African continent more possible. However, power is still a major challenge within Africa. Around half the population have no electricity at home. African governments are calling for support from the Global North via the COP climate summits, recognising the potential for renewable energy generation to support growth in power provision (Payton, 2024). Mitigating these weakness in large scale power infrastructure, there is some hope given that computer devices are increasing in their efficiency. The internet is accessed primarily on low-powered, android-based mobile phones which can be easily recharged on low-cost solar devices. This substantially reduces the material barriers to access AI for educational purposes.
Next, consider the individual digital skills. As mentioned, when considering the motivation to use AI, there are growing digital skills in the younger population, with widespread engagement with technology such as TikTok and YouTube to develop skills. Many young people are also sharing their skills, as creators, monetising and influencing. However, while the digital skills necessary for content generation are quite advanced, consuming content requires less expertise. Therefore, when considering the use of AI in education, there may be a digital skills gap that needs to be addressed. For example, one student may be comfortable using AI on a computer to help construct writing frames for assessment development, whilst another may only be able to use a computer to access social media to watch content. While genAI has a “low barrier” to entry, with a natural language interface, a growing body of research shows that the skill of prompt engineering is required to get the most out of the system (Schulhoff et al., 2024).
Critical evaluation skills must be developed for effective use of AI-generated content. Generative AI does not actually know anything. It is a prediction machine whose output is based on the probability of the next token (a word, or part of a word) being the most likely. It cannot be relied upon for facts. Studies have shown that the output is often wrong as generative AI “hallucinates” (Ji et al., 2023), inventing facts with convincing confidence. It cannot be reliably used for technical education or subjects that rely on technical or regulatory data (Birtill & Birtill, 2024), as it is unable to apply contextual information, which may vary by international setting, effectively. It is also biased. This is because the datasets that Large Language Models (LLMs) are trained on contain biases. These biases reflect the biases on the internet in general (Navigil et al., 2023). For example, Reddit was included in the training data of ChatGPT, which can be an unreliable source as anyone can post to it with their biases (Ravi & Vela, 2024). Additionally, relevant for education, some LLMs have been trained to censor content. For example, ChatGPT flagged a request for material about genocide studies as inappropriate content. It refused to analyse German military documents from 1939, while being able to analyse similarly dated allied documents (Waddington, 2024). Therefore, critical evaluation skills are needed to judge the appropriateness of the generated output.
Finally, consider usage – the degree to which different groups use AI. There is already evidence in the global north of gender differences in usage of AI (Carvajal et al., 2024), with female students being more likely to opt out of its use. While there remain participation gaps in the global south, many of these are driven by the factors discussed above (Udisha & Ambily Philomina 2024). When skills, material access, and motivation are addressed, AI will be more able to support educational needs.
Considering the access framework outlined above, and the analysis of AI in education through this perspective, it appears that AI in education has the potential to succeed. Indeed, early research trials are beginning to emerge that demonstrate the success of AI-supported educational tools. Henkel et al., (2024) in a preprint article describe a trial that took place in Ghana. Rori, an AI-powered maths tutor was made available to students via WhatsApp on a mobile device for only one hour a week. These students showed a substantial improvement in maths, compared to student who did not have access to this tool, but had all other experiences the same. This unstructured access to the AI tool demonstrates the possibility of children engaging with AI as a way of learning that does not rely on direct instruction, aligning with the Vygotskian constructivist approach (Sidorkin, 2024).
The Global South views AI as transformative, aligning with the optimism of big tech in the Global North. However, this is at odds with the extractive, colonial, and unreliable nature of the technology. Algorithmic colonisation is driven by the corporate agenda of companies such as Meta (Facebook, Instagram, WhatsApp etc.) and OpenAI. This colonialism is different to the colonialism of the past that was spatial; however, it is still the same extractive model where those of the Global South may be exploited for the profits of the Global North. In addition, this colonisation still impacts the workers of the Global South, imprisoning the workers and making them dependent on the infrastructure and technology of the Global North. Again, the charisma is sold as a solution to the problems of the South; however, as Birhane (2020) states, Western developed AI solutions may not be suitable for the Global South, may impede the development of local solutions that take account of the specific needs of the Global South.
The problems of technology must be balanced against their perceived usefulness. Allman (2022) points out that digital tools have become woven into society, and access to them should be seen as a right. There is a desire for the use of AI technology in the Global South as a solution to problems of education and economic development. The risks of depending on AI technology to solve deep-rooted institutional, societal and economic problems is high, this along with the human cost and the environmental impact. This could paradoxically lead to greater digital inequality as the resources used for AI generation, whether that be power, data or human capital, could lead to expansion of the North-South digital divide and thereby increasing the socio-economic, developmental and educational problems that the Global South is wishing to solve with AI technology. The rapid expansion of digital tools, and especially AI tools, mirrors classic colonialism. Like in the past when Western countries exploited the Global South, extracting resources for profit in the North by building technical infrastructure to do so. The charismatic, seductive technology of the railways in the late 19th century has been replaced by the technology infrastructure of the 21st century.
If the promise of AI in education that is sold by its proponents – one of automation, liberation and societal development – is to be achieved, then there is required a substantial critical revaluation of the relationship between the companies who own the tools and the workers who train them. New models and relationships need to be developed between the owners of the technology in the North and the trainers in the Global South, along with robust critical and ethical frameworks to ensure that there are fair labour practices together with fair development of AI solutions that benefit the Global South rather than colonise them. Indeed, the Africa Union has stated that they wish to create Africa owned specific AI tools which will be able to address the unique challenges in African education such as linguistic barriers or access to education (Africa Union, 2024).
Our understanding of AI adoption in the Global South needs significant reframing. The narrative must shift from one of technological determinism and Western-centric edtech solutions to one that takes account of local contexts and needs. This requires acknowledging that communities in the Global South are not passive recipients of technology but active agents in shaping how AI can serve their specific needs and development goals. Without stringent ethical frameworks and safeguards around the deployment, training, human costs and use of AI, it is likely that the charismatic extraction machine will continue to increase inequality in the people who pay the largest cost for this technology. This technology must be more than just “mathematical snake oil” (Birhane, 2020). If the wishes of UNESCO, the African Union and others in the Global South are to be met and the optimism of youth fulfilled, then this is an urgent reassessment that needs to take place to ensure that the technological benefits can be harnessed for the benefit of all.
References
Adelana, O. P., Ayanwale, M. A., & Sanusi, I. T. (2024). Exploring pre-service biology teachers’ intention to teach genetics using an AI intelligent tutoring-based system. Cogent Education, 11(1), https://doi.org/10.1080/2331186X.2024.2310976
Africa Union. (2024). Continental Artificial Intelligence Strategy: Harnessing AI for Africa’s Development and Prosperity. Africa Union. https://au.int/en/documents/20240809/continental-artificial-intelligence-strategy
Ahmad, N. & Salvadori, K. (2020, May 13). Building a transformative subsea cable to better connect Africa. Engineering at Meta. https://engineering.fb.com/2020/05/13/connectivity/2africa/
Ali, R., Tang, O. Y., Connolly, I. D., Zadnik Sullivan, P. L., Shin, J. H., Fridley, J. S., … & Telfeian, A. E. (2023). Performance of ChatGPT and GPT-4 on neurosurgery written board examinations. Neurosurgery, 93(6), 1353-1365. https://doi.org/10.1101/2023.03.25.23287743
Allmann, K. (2022). UK digital poverty evidence review 2022. London: Digital Poverty Alliance. https://digitalpovertyalliance.org/uk-digital-poverty-evidence-review-2022/
Ames, M. G. (2015, August). Charismatic technology. In Proceedings of the fifth decennial Aarhus Conference on Critical Alternatives (pp. 109-120). https://doi.org/10.7146/aahcc.v1i1.21199
Ames, M. G. (2019). The charisma machine: The life, death, and legacy of one laptop per child. Mit Press.
Arora, P. (2010). Hope‐in‐the‐Wall? A digital promise for free learning. British Journal of Educational Technology, 41(5), 689-702. https://doi.org/10.1111/j.1467-8535.2010.01078.x
Arora, P. (2024a). The privilege of pessimism: The politics of despair towards the digital and the moral imperative to hope. Dialogues on Digital Society, 0(0). https://doi.org/10.1177/29768640241252103
Arora, P. (2024b). Creative data justice: a decolonial and indigenous framework to assess creativity and artificial intelligence. Information, Communication & Society, 1-17. https://doi.org/10.1080/1369118X.2024.2420041
Birhane, A. (2020). Algorithmic colonization of Africa. SCRIPTed, 17, 389. http://dx.doi.org/10.2966/scrip.170220.389.
Birtill, M., & Birtill, P. (2024). Implementation and Evaluation of Genai-Aided Tools in a UK Further Education College. In Artificial Intelligence Applications in Higher Education (pp. 195-214). Routledge. http://dx.doi.org/10.4324/9781003440178-12
Carvajal, D., Franco, C., & Isaksson, S. (2024). Will Artificial Intelligence Get in the Way of Achieving Gender Equality?. NHH Dept. of Economics Discussion Paper, (03). http://dx.doi.org/10.2139/ssrn.4759218
Department for Education. (2023, October 30). New support for teachers powered by Artificial Intelligence [Press release]. https://www.gov.uk/government/news/new-support-for-teachers-powered-by-artificial-intelligence
Department for Education. (2024, August 28). Teachers to get more trustworthy AI tech, helping them mark homework and save time [Press release].https://www.gov.uk/government/news/teachers-to-get-more-trustworthy-ai-tech-as-generative-tools-learn-from-new-bank-of-lesson-plans-and-curriculums-helping-them-mark-homework-and-save
Doroudi, S. (2023). The intertwined histories of artificial intelligence and education. International Journal of Artificial Intelligence in Education, 33(4), 885-928. https://doi.org/10.1007/s40593-022-00313-2
Ebadi, S., & Amini, A. (2022). Examining the roles of social presence and human-likeness on Iranian EFL learners’ motivation using artificial intelligence technology: A case of CSIEC chatbot. Interactive Learning Environments, 32(2), 655-673. https://doi.org/10.1080/10494820.2022.2096638
Eke, D. O. (2023). ChatGPT and the rise of generative AI: Threat to academic integrity?. Journal of Responsible Technology, 13. https://doi.org/10.1016/j.jrt.2023.100060
Henkel, O., Horne-Robinson, H., Kozhakhmetova, N., & Lee, A. (2024). Effective and Scalable Math Support: Evidence on the Impact of an AI-Tutor on Math Achievement in Ghana. arXiv preprint. https://doi.org/10.48550/arXiv.2402.09809
Gartner. (2024, August 21). Gartner 2024 Hype Cycle for Emerging Technologies Highlights Developer Productivity, Total Experience, AI and Security. [Press release]. https://www.gartner.com/en/newsroom/press-releases/2024-08-21-gartner-2024-hype-cycle-for-emerging-technologies-highlights-developer-productivity-total-experience-ai-and-security
Gethin, A. (2023). Distributional Growth Accounting: Education and the Reduction of Global Poverty, 1980-2022. World Inequality Lab
Giannini, S. (2023). Generative AI and the future of education. UNESCO. https://doi.org/10.54675/HOXG8740
Ji, Z., Lee, N., Frieske, R., Yu, T., Su, D., Xu, Y., … & Fung, P. (2023). Survey of hallucination in natural language generation. ACM Computing Surveys, 55(12), 1-38. https://doi.org/10.1145/3571730
Kamalov, F., Santandreu Calonge, D., & Gurrib, I. (2023). New era of artificial intelligence in education: Towards a sustainable multifaceted revolution. Sustainability, 15(16), https://doi.org/10.3390/su151612451
Kasun, G. S., Liao, Y. C., Margulieux, L. E., & Woodall, M. (2024). Unexpected outcomes from an AI education course among education faculty: Toward making AI accessible with marginalized youth in urban Mexico. In Frontiers in Education, 9(13), https://doi.org/10.3389/feduc.2024.1368604
Katz, D. M., Bommarito, M. J., Gao, S., & Arredondo, P. (2024). Gpt-4 passes the bar exam. Philosophical Transactions of the Royal Society A, 382(2270), https://doi.org/10.1098/rsta.2023.0254
Kestin, G., Miller, K., Klales, A., Milbourne, T., & Ponti, G. (2024). AI Tutoring Outperforms Active Learning. Preprint. https://doi.org/10.21203/rs.3.rs-4243877/v1
Khlaif, Z. N., Ayyoub, A., Hamamra, B., Bensalem, E., Mitwally, M. A., Ayyoub, A., … & Shadid, F. (2024). University Teachers’ Views on the Adoption and Integration of Generative AI Tools for Student Assessment in Higher Education. Education Sciences, 14(10). https://doi.org/10.3390/educsci14101090
Linxen, S., Sturm, C., Brühlmann, F., Cassau, V., Opwis, K., & Reinecke, K. (2021). How weird is CHI?. In Proceedings of the 2021 chi conference on human factors in computing systems (pp. 1-14). https://doi.org/10.1145/3411764.3445488
Mei, Q., Xie, Y., Yuan, W., & Jackson, M. O. (2024). A Turing test of whether AI chatbots are behaviorally similar to humans. Proceedings of the National Academy of Sciences, 121(9), e2313925121. https://doi.org/10.1073/pnas.2313925121
Miao, F., Holmes, W., Huang, R., & Zhang, H. (2021). AI and education: A guidance for policymakers. Unesco.
Mitra, S., & Rana, V. (2001). Children and the Internet: Experiments with minimally invasive education in India. British Journal of Educational Technology, 32 (2), 221-232. https://doi.org/10.1111/1467-8535.00192
Memarian, B., & Doleck, T. (2023). ChatGPT in education: Methods, potentials and limitations. Computers in Human Behavior: Artificial Humans, 100022. https://doi.org/10.1016/j.chbah.2023.100022
Merchant, B. (2024). AI generated business: The rise of AGI and the rush to find a working revenue model. AI Now Institute. Retrieved December 5, 2024, from https://ainowinstitute.org/general/ai-generated-business
Muldoon, J., Graham, M., & Cant, C. (2024). Feeding the machine: the hidden human labour powering AI. Canongate Books.
Navigli, R., Conia, S., & Ross, B. (2023). Biases in large language models: origins, inventory, and discussion. ACM Journal of Data and Information Quality, 15(2), 1-21. https://doi.org/10.1145/3597307
Payton, B. (2024, October 18). Can Africa hit the accelerator on renewables? African Business. https://african.business/2024/10/energy-resources/can-africa-hit-the-accelerator-on-renewables
Pedro, F., Subosa, M., Rivas, A., & Valverde, P. (2019). Artificial intelligence in education: Challenges and opportunities for sustainable development. UNESCO.
Ravi, K., & Vela, A. E. (2024). Comprehensive dataset of user-submitted articles with ideological and extreme bias from Reddit. Data in Brief, 56, 110849. https://doi.org/10.1016/j.dib.2024.110849
Rospigliosi, P. A. (2023). Artificial intelligence in teaching and learning: What questions should we ask of ChatGPT? Interactive Learning Environments, 31(1), 1-3. https://doi.org/10.1080/10494820.2023.2180191
Rowe, N. (2023, August 2). ‘It’s destroyed me completely’: Kenyan moderators decry toll of training of AI models. The Guardian. https://www.theguardian.com/technology/2023/aug/02/ai-chatbot-training-human-toll-content-moderator-meta-openai
Schulhoff, S., Ilie, M., Balepur, N., Kahadze, K., Liu, A., Si, C., … & Resnik, P. (2024). The Prompt Report: A Systematic Survey of Prompting Techniques. arXiv preprint https://doi.org/10.48550/arXiv.2406.06608
Schiff, D. (2022). Education for AI, not AI for education: The role of education and ethics in national AI policy strategies. International Journal of Artificial Intelligence in Education, 32(3), 527-563. https://doi.org/10.1007/s40593-021-00270-2
Selwyn, N. (2019). Should robots replace teachers?: AI and the future of education. John Wiley & Sons.
Sidorkin, A. M. (2024). Artificial intelligence: Why is it our problem? Educational Philosophy and Theory, 1–6. https://doi.org/10.1080/00131857.2024.2348810
Slagg, A. (2023, November 14). AI for Teachers: Defeating Burnout and Boosting Productivity. EdTech Magazine. https://edtechmagazine.com/k12/article/2023/11/ai-for-teachers-defeating-burnout-boosting-productivity-perfcon
Strielkowski, W., Grebennikova, V., Lisovskiy, A., Rakhimova, G., & Vasileva, T. (2024). AI‐driven adaptive learning for sustainable educational transformation. Sustainable Development. https://doi.org/10.1002/sd.3221
The Global Goals. (n.d.). 4 Quality Education. Retrieved December 1, 2024, from https://www.globalgoals.org/goals/4-quality-education/?gad_source=1&gclid=Cj0KCQiA3sq6BhD2ARIsAJ8MRwWcfHxh4hBRi-MwyrMdU7HsFj8oTkS2GroXqtlw-XfnpQ17sTlYWt0aApsrEALw_wcB
Tucker, I. (2024, July 6). James Muldoon, Mark Graham and Callum Cant: ‘AI feeds off the work of human beings.’ The Observer. https://www.theguardian.com/technology/article/2024/jul/06/james-muldoon-mark-graham-callum-cant-ai-artificial-intelligence-human-work-exploitation-fairwork-feeding-machine
Udisha, O., & Ambily Philomina, I. G. (2024). Bridging the Digital Divide: Empowering Rural Women Farmers Through Mobile Technology in Kerala. Sustainability, 16(21), 9188. https://doi.org/10.3390/su16219188
UNESCO. (2019). Exploring the potential of artificial intelligence to accelerate the progress towards SDG 4 -Education 2030. UNESCO Executive Board, 206th, 2019 [587]
Van Dijk, J. (2020). The digital divide. John Wiley & Sons.
Waddington, L. (2024), November 25). Navigating Academic Integrity in the Age of GenAI: A Historian’s Perspective on Censorship. International Center for Academic Integrity. https://academicintegrity.org/resources/blog/536-navigating-academic-integrity-in-the-age-of-genai-a-historian-s-perspective-on-censorship
Wall, P. J., Saxena, D., & Brown, S. (2021). Artificial intelligence in the Global South (AI4D): Potential and risks. arXiv preprint arXiv:2108.10093. https://doi.org/10.48550/arXiv.2108.10093
Wang, R. E., Ribeiro, A. T., Robinson, C. D., Loeb, S., & Demszky, D. (2024). Tutor copilot: A human-ai approach for scaling real-time expertise. arXiv preprint https://doi.org/10.48550/arXiv.2410.03017
Zawacki-Richter, O., Marín, V. I., Bond, M., Gouverneur, F., & Hunger, I. (2019). Systematic review of research on artificial intelligence applications in higher education—where are the educators? International Journal of Educational Technology in Higher Education, 16(1), 39. https://doi.org/10.1186/s41239-019-0171-0
AI in UK Further Education
Artificial intelligence (AI) can be defined as “machines that can complete cognitive tasks” (Brynjolfsson & McAfee, 2014 p. 91). Recent developments in generative AI, which creates texts from prompts using large language models trained on vast amounts of text data, have prompted a re-examination of the use of AI in education (Cotton et al., 2023). The launch of OpenAI’s ChatGPT in October 2022 has particularly driven conversation due to its ease of use and conversation style. Claims have been made that it can pass several accredited exams including UK medical exams (Maitland et al., 2024). However, the use of AI tools is not new to education. AI has been embedded in tools used by educators for many years (Zawacki-Richter et al., 2019). For example, spell checking in Microsoft Word is based on AI. In wider society, Apple’s Siri and Amazon’s Alexa are examples of AI-based digital assistants. JISC (2024a) claim that generative AI has the power to transform education, and commercial companies such as Teachermatic have produced ChatGPT-based tools that offer educators tools to produce educational content such as multiple-choice quizzes, lesson plans, and marking rubrics, which they claim will save teacher time and reduce teacher workload (Sheppard, 2023).
In addition, this technology has many possible benefits for students. For example, it could help with accessibility, organise work, offer suggestions for starting points, compose writing frames, and generate plausible content. Indeed, one academic paper, Cotton et al., (2023), used ChatGPT to generate a large proportion of the paper.
The reaction in education has been mixed. On one hand, some educators have embraced the opportunities offered by generative AI (Meakin, 2024), while others are sceptical (Selwyn, 2024). There has been much discussion around the use of AI tools by students completing assessments and whether this use amounts to academic malpractice. This needs to be considered in the light of the fact that 58% (FE News, 2023) of students in a survey reported using ChatGPT. However, the survey also pointed out that students were using ChatGPT as a study aid rather than a straightforward plagiarism tool, and only 4% of those surveyed admitted that they copy and pasted material directly from chatGPT.
Technological solutions for detecting student use of GenAI have been launched (Turnitin, n.d.), with some educators and exam bodies relying on the technology to detect academic misuse of the tools. However, it has been shown that this technology is unreliable, produces false positives (Weber-Wulff et al., 2023), and is discriminatory towards those who have English as a second language (Rospigliosi, 2023), irrespective of the claims made by the manufacturers of these tools. There has been a mixed reception to these tools by educational establishments, with some relying on it (University of Lincoln, 2024), while others have turned it off (McMahon & Hutt, 2023).
Within the education sector in the UK, much advice has been given to both the school sector and the higher education sector. The Russell Group (2023) has produced guidance for universities which takes a progressive stance on AI adoption and use; however, at the time of writing, no such guidance has been produced by the Association of Colleges for the FE sector.
As a manager in FE, I want to understand how to support my college in responding appropriately to these generative AI developments. I want to understand the feelings of, and impact on teachers of GenAI. The implementation of new technology in educational settings can be complex. UNESCO state that pedagogy can be transformed by the integrating and accepting digital technologies within education (UNESCO, 2018). This can only be achieved if the digital literacy of the educators and the technical resources of the institution are sufficient. Training in digital use has only been recently introduced in initial teacher training in FE (ETF, 2023) and as Bećirović (2023) points out, many teachers’ digital literacy and competence has not developed beyond the level from their own training. The successful integration of digital tools in education is often due to the enthusiasm of the individual for digital technology (Beetham & Sharpe, 2023).
In considering the impact of AI on FE, I will use the Technology Acceptance model (Davis 1989). This considers the factors which impact technology acceptance and is derived from both the theory of reasoned action and the theory of planned behaviour. Granić & Marangunić, (2019) state that perceived usefulness is the key factor underlying technology acceptance, which in turn is affected by the perceived ease of use. Generative AI is a disruptive innovation in education, challenging our existing practices and systems. Bowyer and Christianson’s (1995) theory of disruptive innovation suggests that incumbents often fail to exploit innovation. As a relatively newly formed college group, there is the opportunity to embrace GenAI to improve the delivery of education to our community.
There is little academic literature to date evaluating the impact of AI from a manager’s perspective. Therefore, I will analyse the guidance for FE given to educators in my college group. I will assess the guidance tensions, agreements, and their relevance as guidance for my staff as an FE manager. It is appropriate start with an analysis of the guidance as this has not yet been extensively implemented in any context, because of how new the technology is, and how slow institutional responses have been. Considering whether the guidance from different stakeholders aligns is important, to examine whether advice is consistent.
The key advice given to staff in our college group are the JISC guide: Principles for the use in FE colleges (JISC, 2024b), and JCQ AI Use in Assessment: Protecting the Integrity of Qualifications (JCQ, 2024). JISC is the UK agency for digital and technical support in further and higher education. They conduct research, provide advice, and offer digital services for these sectors. JISC leads on the digital transformation of further and higher education in the UK. JCQ is the Joint Council for Qualifications, representing the major awarding bodies in the UK. They are primarily concerned with maintaining examination integrity through policy and standardisation of exam procedures. As such this advice and guidance may not be consistent as they are trying to achieve different goals.
I will now consider the guidance, first examining the guidance regarding staff using AI to support their own work, and then looking at the guidance regarding staff supporting students use of AI tools.
The JISC advice takes an innovative approach to the use of AI. It looks at how staff may use the tool to support their own work. It discusses the limitations and biases of the technology and suggests that students should be informed when AI is used to create learning resources or in supporting marking.
“Education institutions should also be open and transparent, ensuring the learners understand when AI is used to create learning resources, support learning delivery or within the assessment and monitoring process. Learners will also be informed how their personal data is being processed using AI tools.” (JISC, 2024b. 1.2)
It discuses that there seems to be some evidence for the tools reducing workload by automating some educational tasks.
“Alongside making tasks quicker, activities that were challenging before because of time constraints become possible. Examples include improved differentiation for learners, using AI to create resources in multiple ways and using AI to create formative assessment resources and materials.” (JISC, 2024b. 3.1)
The guidance seeks to set out fair and open approach to AI use, whilst acknowledging that the use of AI is only going to increase, not only in education but the wider world and places the responsibly on colleges to equip students with the required skills to progress in an AI enabled world.
“AI is evolving at a rapid pace, and therefore, while teaching learners to use the AI tools of today is valuable, this needs to be supplemented to include a broader AI literacy, to enable learners to critically evaluate tools of the future.” (JISC, 2024b. 2.1)
The guidance also points appropriate use of AI in assignments – it places the responsibility on colleges of ensure that students are informed about what constitutes appropriate AI use for any assignment.
“All colleges will provide clear guidance to learners on appropriate use of AI in their assignments. This includes general principles and guidance, along with more specific guidance at assessment level.” (JISC, 2024b. 5.2)
It discusses the use of AI detection tools but warns that these tools can be discriminatory and provide false positives when used. As such, it suggests that users of these tools in colleges should be trained in understanding the limitations of AI detection tools.
“There is a risk AI detection can unfairly discriminate and can compound existing bias.” (JISC, 2024b. 5.3)
However, the guidance does suggest that assignments should be made relevant for an AI world, and that colleges should be designing assignments with this in mind and looking at ways that authentic assessment can be maintained whilst ensuring that students develop the necessary AI skills.
“…help them become AI Citizens equipped to navigate the use of AI in their everyday lives.” (JISC, 2024b 2.3)
“…move towards a consistent approach for the use of AI in assessments, with the aim of making assessments authentic and relevant to an AI enhanced workplace and society …” (JISC, 2024b 2.4)
The JCQ guidance is concerned about maintaining the integrity of assessments. Its guidance is concerned about AI use as malpractice in assessments. It does not acknowledge that AI can assist in the development of skills and knowledge and clearly states that students must not rely on AI tools to help them develop knowledge and skills. The guidance emphasises that students work must be their own without AI assistance and warns against using AI generated content as it may be inaccurate or generate false information such as references. There is an acknowledgement that AI might be used in the earlier stages of course work but it doesn’t give clear guidance on the exact extent of what it considers reasonable. It does, however, echo the JISC guidance on being aware of the potential biases of AI generated content and makes emphasise that AI generated information may be incorrect.
The general message coming from the JCQ guidance is to restrict the use of AI. This is contrast to JISC which looks at way to develop the integration of AI into assessment. This restriction is further enhanced by the suggestion that AI tools should be used to detect possibility use of AI in submitted work together with a range of indicators of AI use. However, whilst JCQ suggest that this is part of a holistic approach to detecting AI use, it has been criticised by Advance HE’s Charles Knight (2024) as “vibes” and whilst indicators such as inconsistent grammar, spelling or language use could be caused by the use of an AI tool it isn’t conclusive proof that a tool has been used. This can lead to false accusations of AI use and could discriminate against students with lower standards of English proficiency (Perkins et al., 2023).
The emphasis on the use of automatic detection tools, by most notably, Turnitin and others is again misplaced. Even though is it is suggested with much caution by JISC in their guidance, there has been subsequent further guidance where JISC discourages its use as “institutions therefore shouldn’t rely on AI detection” (Webb, 2023b). Furthermore, studies into the use of AI detection tools confirm this. Weber-Wulff et al., (2023) state that both publicly available and commercial detection tools are “neither accurate nor reliable.” Price & Sakellarios (2023) warn that AI detectors will generate more false positives to non-native English speakers, than native ones. While Sadasivan et al, (2024) point out that AI detection can be broken by paraphrasing the generated text.
The two documents approach to educators’ use of AI also differs in tone. It is encouraged in the JISC guidance. It acknowledges the possibilities that AI can offer and actively encourages the discussion on how colleges can integrate these tools in their teaching and assessment, whilst at the same time ensuring that students are prepared for an AI enabled world. The JCQ document however sees AI use as a risk to assessments. It places the emphasis on the educator’s responsibility to ensure that students understand the JCQ stance on what constitutes AI misuse. JCQ believe that students must not use AI tools in the production of assessed work. There is no discussion of how AI could be integrated into assessment practice to produce better, more relevant assessment as the validity of the assessment is related to their authenticity. Understanding AI use as merely a risk diminishes the educational gain that might be achieved when incorporating AI.
As guidance for educators and mangers within my institution, these documents, whilst containing inherent contradictions, make the possibilities of AI adoption problematic. As a manager I wish to incorporate AI technologies within the department. The possibilities of AI to enhance learning are great, however due to rapidly changing landscape of AI use and adoption guidance for staff should help them navigate the change to an AI enabled world.
The JISC document is helpful as it gives guiding principles to use when considering integrating AI into the curriculum. The JCQ documents gives rules to be adhered to when conducting existing currently designed assessments. AI is a disruptor to current assessment practices, and as such this is point where we need to consider what assessment is for and how does it represent the skills required by students within my sector. Assessments need to be valid and authentic for an AI enabled world. However, there is no discussion around this coming from JCQ as the representative of awarding bodies.
Within my department, most of my students are apprentices or preparing for work in engineering. The wider engineering workplace is rapidly adopting AI and students need to be equipped with AI skills (UKESF, 2023). Students need to understand what AI is doing in their specific area of engineering. The world is being rapidly AI enabled and students need to have the tools to navigate the rapid developments. This aligns with the JISC guidance and is a progressive approach to AI adoption in education.
The engineering apprenticeship is assessed in several different ways. Direct observation and professional discussions in the workplace are clearly AI-proof, as the apprentice is actively working in the environment, producing, or repairing engineering machinery. This method is reliable and authentic for assessment. Apprentices also must sit short-answer paper exams under exam conditions and online multiple-choice question papers, again under JCQ exam conditions. These are AI-proof assessments as they assess the candidates recall of knowledge under traditional exam conditions.
The area where AI may be used is in the production of job reports. Within the apprenticeship, for every assessment, the candidate must produce a reflective technical account relating to a task for which they have been examined. This is to contextualize learning at college within their specific engineering practice. This is an area where generative AI could be used to produce the report, which could be seen as plagiarism in line with JCQ guidance. Conversely, generative AI could be used to help the student produce a better-quality written report, and help them frame, explore, and develop their reflective technical account. However, this would then be outside the scope of the JCQ guidance.
The issues raised in the JCQ document concerning assessment need to be addressed. As pointed out, there is concern about the use of generative AI in summative assessments. Plagiarism by use of generated text within a submitted assessment is described in the document. The use of generated text is not allowed in these assessments.
When asking Chat GPT 3.5 a question from the level 3 OAL Food & Drink Engineering portfolio, the response produced would fail due to technical errors and not fulfilling the learning outcomes. Chat GPT 3.5 had not been trained on the specific technical data required and therefore cannot produce realistic content; this aligns with the findings of Ji et al. (2023). Hence the use of AI here is insufficient to meet the learning outcome. Therefore, there is little use for AI used in this manner. The JCQ rules do not really have an effect as the assessment would fail due to the content irrespective of who or what produced it.
However, a better use of AI would be to help formulate a writing frame or a starting point for students to develop their work from. This would be useful and allows the student to think about the writing in a logical way. It can also support students who have difficulty writing, with support for spelling and grammar. As an example, I have produced this in the appendix.
However, this represents a grey area in the JCQ documents; the AI is not being used to generate the content – the student needs to provide the correct technical information but is supported in presenting the information in a clear and logical way. This is an appropriate use of the technology. The student will have to have the knowledge, and as such, the assessment is valid and an authentic representation of their knowledge. The AI has been used to support the structure and the English language. As we are assessing for technical knowledge, its use should not present accusations of AI plagiarism.
Staff also need training in digital and AI skills; indeed, this is now being included in the initial teacher training specifications in England (Department for Education, 2024). However, staff in post may have digital literacy training needs. These should be addressed by institutions. At my college, a huge emphasis has been placed on the digital expectations of teachers. Courses are digitally enhanced using the VLE, and the focus is to use this extensively in future. Staff will need to have the required skills to allow this, and training programs are being developed in line with JISC recommendations.
Generative AI can help teachers with producing content. Studies have shown that gen AI tools have the potential to automate the creation of teaching materials, marking, lesson planning, etc., thereby saving time and reducing workload. The UK government has invested £2 million to provide AI resources to educators such as lesson planning, report writing, and content creation (GOV. UK, 2023). The direction from government is to support the progressive use of AI tools within education.
Next, I will gather and analyse qualitative evidence from FE educators on their knowledge and attitudes towards AI usage, evaluating the usefulness of provided guidance and comparing it with my own viewpoint. It is important take in account the lived experience of skilled and experienced educators, as they navigate this new technology. It is interesting to see if the guidance aligns with educators’ viewpoints and is useful to them. As a manager I need to produce supportive guidance that is meaningful to my staff and aligns with their values. Therefore, considering qualitative responses to both AI, and the guidance, will allow me to investigate the best way of supporting my staff in navigating AI.
Methodology
There were two different phases of data collection. The first was part of an evaluation of a GenAI tool based on Chat GPT 3.5, Teacher Matic which includes views on AI and AI tools generally. The second was specifically considering educators response to the JISC and JCQ guidance.
In the first collection of data, 22 staff (9 females) gave short answers to an online survey. Participants provided consent. Demographic data such as their age, gender, and how long they had been teaching was gathered. They were asked open ended questions about their prior use of digital pedagogical tools and their familiarity and experience with generative AI technologies. Finally, they were asked five open ended questions about the AI tool. These questions aimed to obtain initial perceptions and potential biases about the use of AI in education.
The second phase of qualitative data collection was conducted by sending questions to teaching staff, staff developers and the Director of Quality, about the JISC and JCQ guidance. A deductive analytical approach was taken, examining alignment between the values of the staff and the guidance that has been issued.
I analysed the data using reflexive thematic analysis (RTA, Braun & Clarke, 2021). RTA can help understand the thoughts and feelings of educators in depth and as such is an appropriate analytical approach. It allows for the bias and perspective that the researcher brings to the process. While also being flexible and allowing the capture of the themes of perceived ease of use and usefulness which are relevant to TAM. It also allows the capture of what educators think about AI and why they think that way.
Findings
Four themes were identified in the qualitative responses – positive attitudes to technology, can we trust GenAI, students are already using AI, and impact on assessment.
Theme 1. Positive attitudes to technology
Educators were generally positive towards the adopting of AI technology with views ranging from the entirely enthusiastic “I love it” to acknowledging the potential benefits but requiring “a clear standardised policy”. A common positive was that the hope that the technology would aid the production of teaching material and planning, and the reduction of workload. One respondent said, “it can help to plan more interesting exercises”, and “swiftly create lesson plans and learning activities”. Another highlighted the positive benefits of AI in education saying it can help with “personalised training, feedback and improved assessment processes.” There is clearly a desire for using the AI technology and its potential usefulness. There is a clear wish for it to be incorporated as an additional tool to help with the teaching an assessment process.
Theme 2. Can we trust GenAI?
This theme was evident in the responses from both data sets. Concerns were raised over the accurucay and correct responses of the AI generated text with one respondent noting that the “information can sometimes be incorrect, and it is important to remember this.” While another stated that the “content requested was not current legislation”. Another was similarly concerned over the sources of information and their accuracy “I am still unsure where the information these platforms have gained from and is it correct?”
Another area of trust was around the ethical implications of the content created such as the “potential risk associated with its use, particularly deep fakes and ownership “. Whilst another educator pointed out that we need to be concerned “about the biases in AI algorithms.”
Theme 3. Students are already using AI
Educators were aware that students are using AI: “students are increasingly using AI in various aspects of their academic lives”. The use of AI was not just limited to the production of study aids or as research tool. Some students had been running their assessment work through AI to “make it sound cleverer” whilst claiming by doing this that they would “learn bigger words”. Whilst others had been subjected to malpractice allegations due generating content for assessment. It is interesting to note that in several cases this was in students who did not have English as a first language, and this could have been due to the biases of the AI detection software in line with the findings of Price & Sakellarios (2023).
Theme 4. Impact on assessment.
In this theme there was some discussion over the impact on assessment, with one respondent welcoming the JCQ guidance as “baseline guidance that can be applied to the context of a variety of assessments” and “guidance on how to inform, guide and tackle around misuse”. Others thought that AI would impact assessment integrity due to the “possibility of cheating facilitated by AI-powered tools.”
However, others thought that AI would impact on the nature of assessment and that this could “inspire teams to look at authentic assessment in more depth rather than simply doing what they’ve always done.” Going beyond this one respondent noted that “I think the way we assess is wrong“ and that “we need to focus on skills and behaviours development”. This reassessment due to AI of what assessment is for and why assess was seen as a good thing as it “should make awarding bodies and educators rethink what we are actually assessing”.
Throughout the themes there is an acceptance of AI. The entry to using AI is low and consequently easy due to AI generators being readily accessible to both students and staff. The adoption of AI use is therefore due its ease of use and usefulness in creating content. This would indicate that acceptance of AI as a new technology is in line with TAM.
Conclusion
As a manager, I cannot stop the march of AI. Instead, we will need to critically consider how and why we assess students. We need to reconsider the purpose of assessment. I can see the educational possibilities of AI for both staff and students. Within my disciplines, many of the practical aspects of the work will not be immediately affected by AI. We will need to ensure we are assessing process – something which is already a key part of vocational qualifications and have less of a reliance on assessment of artefacts for valid and reliable assessment. AI is the disruptor of education assessment hegemony. However, whenever there is disruption there is also opportunity. As pointed out by Brynjolfsson & McAfee (2011) we need to learn to “race with the machines” rather than fight them. Our focus should be on skills and behaviours development rather than regurgitation of facts. The punitive JCQ rules whilst being guidance for existing assessments that may be appropriate for A level written assessments are not suitable for the wider needs of vocational education where skills fluency is a more appropriate metric.
My students will be working in an AI enabled world. Without teaching them the critical thinking skills necessary to engage with and analyse the output of AI, this will impact their ability to work effectively in their disciplines. As Ji et al, (2023) have pointed out, genAI often produces incorrect information. However, within engineering it is imperative that precise information is provided as it is often safety critical. As such critical thinking skills should be taught and reinforced to help students understand that they must assess whether the output given from AI generation is correct. AI education needs to be placed into the wider context of ethical use to ensure that students are taught about the inherent biases with the AI due to the how, where and by who it has been trained (Webb, 2023a).
AI challenges us to move from the assessment and learning methods of the past and move to ones that are relevant for the future UK as a global AI superpower (DDCMS, 2021).
References
Bećirović, S. (2023). Digital Competence of Teachers and Students. In S. Bećirović (Ed.), Digital Pedagogy (pp. 39-50). Springer Briefs in Education. Springer. https://doi.org/10.1007/978-981-99-0444-0_4
Beetham, H., & Sharpe, R. (Eds.). (2019). Rethinking Pedagogy for a Digital Age: Principles and Practices of Design (3rd ed.). Routledge. https://doi.org/10.4324/9781351252805
Bower, J. L., & Christensen, C. M. (1995). Disruptive technologies: Catching the wave. Harvard Business Review, 73(1), 43–53.
Brynjolfsson, E., & McAfee, A. (2011). Race against the machine: How the digital revolution is accelerating innovation, driving productivity, and irreversibly transforming employment and the economy. Digital Frontier Press.
Brynjolfsson, E., & McAfee, A. (2014). The second machine age: Work, progress, and prosperity in a time of brilliant technologies. WW Norton & Company.
Cotton, D. R. E., Cotton, P. A., & Shipway, J. R. (2023). Chatting and cheating: Ensuring academic integrity in the era of ChatGPT. Innovations in Education and Teaching International. https://doi.org/10.1080/14703297.2023.2190148
Davis, F. D. (1989). Perceived usefulness, perceived ease of use, and user acceptance of information technology. MIS Quarterly, 13(3), 319 –339.
Department for Digital, Culture, Media & Sport. (2021, September 22). New ten-year plan to make the UK a global AI superpower. [Press release]. https://www.gov.uk/government/news/new-ten-year-plan-to-make-britain-a-global-ai-superpower
Department for Education. (2023). Generative artificial intelligence (AI) in education. https://www.gov.uk/government/publications/generative-artificial-intelligence-in-education/generative-artificial-intelligence-ai-in-education
Department for Education. (2023, May 7). Diploma in Teaching (Further Education and Skills) qualification framework. https://www.gov.uk/government/publications/further-education-initial-teacher-education/diploma-in-teaching-further-education-and-skills-qualification-framework
ETF (2023). Qualification framework for the full teaching qualification for the further education and skills sector https://www.et-foundation.co.uk/wp-content/uploads/2024/01/Qualification-Framework-for-Diploma-in-Teaching-FES-Dec23-update.pdf
FE News. (2023, March 13). PebblePad survey finds UK students using AI tools to support studies, not to outsource writing. https://www.fenews.co.uk/student-view/pebblepad-survey-finds-uk-students-using-ai-tools-to-support-studies-not-to-outsource-writing/
GOV.UK (2023, October 30). New support for teachers powered by Artificial Intelligence. https://www.gov.uk/government/news/new-support-for-teachers-powered-by-artificial-intelligence#:~:text=The%20Government%20is%20investing%20up,personalised%20AI%20lesson%2Dplanning%20assistant.
Granić, A., & Marangunić, N. (2019). Technology acceptance model in educational context: A systematic literature review. British Journal of Educational Technology, 50(5), 2572–2593. https://doi.org/10.1111/bjet.12864
Ji, Z., Lee, N., Frieske, R., Yu, T., Su, D., Xu, Y., Ishii, E., Bang, Y., Madotto, A., & Fung, P. (2023). Survey of hallucination in natural language generation. ACM Computing Surveys, 55(12), 1-38. https://doi.org/10.1145/3571730
JISC. (2024a) Generative AI – a primer. Version 1.3. https://repository.jisc.ac.uk/9407/1/Jan_2024_generative_AI_primer-final.pdf
JISC. (2024b). Principles for the use of AI in FE colleges. https://www.jisc.ac.uk/further-education-and-skills/principles-for-the-use-of-ai-in-fe-colleges
Joint Council for Qualifications. (2024). Artificial Intelligence (AI) Use in Assessments: Protecting the Integrity of Qualifications. https://www.jcq.org.uk/wp-content/uploads/2024/04/AI-Use-in-Assessments_Feb24_v6.pdf
Knight, C., [@Charlesknight]. (2024, April 5) The more I think about it – it’s wild that people think ensuring academic integrity in an age of AI comes down to individuals using “vibes”. https://twitter.com/Charlesknight/status/1776155966523752526
Maitland, A., Fowkes, R., & Maitland, S. (2024). Can ChatGPT pass the MRCP (UK) written examinations? Analysis of performance and errors using a clinical decision-reasoning framework. BMJ Open, 14, e080558. https://doi.org/10.1136/bmjopen-2023-080558
McMahon, M., Hutt, I. (2023). Use of AI (Artificial Intelligence) detection tools in Turnitin. https://www.staffnet.manchester.ac.uk/bmh/about-fbmh/news-and-events/news/display/?id=29633
Meakin, L, A. (2024) Embracing Generative AI in the Classroom Whilst Being Mindful of Academic Integrity. In S. Mahmud (Ed), Academic Integrity in the Age of Artificial Intelligence. IGI Global. https://doi.org/10.4018/979-8-3693-0240-8.ch004
OAL. (2018). Level 3 Diploma in Food and Drink Engineering Maintenance 603/2374/7. Qualification Handbook. Occupational Awards Limited.
OpenAI. (2024). ChatGPT (May 1 version) [Large language model]. https://chat.openai.com/chat
Perkins, M., Roe, J., Postma, D., McGaughran, J., & Hickerson, D. (2024). Detection of GPT-4 Generated Text in Higher Education: Combining Academic Judgement and Software to Identify Generative AI Tool Misuse. Journal of Academic Ethics 22, 89–113. https://doi.org/10.1007/s10805-023-09492-6
Price, G., & Sakellarios, M. (2023). The Effectiveness of Free Software for Detecting AI-Generated Writing. International Journal of Teaching, Learning and Education, 2, 31-38. https://doi.org/10.22161/ijtle.2.6.4
Rospigliosi, P. A. (2023). Artificial intelligence in teaching and learning: What questions should we ask of ChatGPT? Interactive Learning Environments, 31(1), 1-3. https://doi.org/10.1080/10494820.2023.2180191
Russell Group. (2023, July 4). New principles on use of AI in education. https://russellgroup.ac.uk/news/new-principles-on-use-of-ai-in-education/
Sadasivan, V. S., Kumar, A., Balasubramanian, S., Wang, W., & Feizi, S. (2024). Can AI-Generated Text be Reliably Detected? arXiv. https://arxiv.org/abs/2303.11156
Selwyn, N. (2024). Constructive Criticism? Working with (Rather than Against) the AIED Back-Lash. International Journal of Artificial Intelligence in Education 34, 84–91. https://doi.org/10.1007/s40593-023-00344-3
Sheppard, P., (2023, August 8). TeacherMatic Pilot – Using generative AI to reduce workload. JISC Artificial intelligence. https://nationalcentreforai.jiscinvolve.org/wp/2023/08/08/teachermatic-pilot-using-generative-ai-to-reduce-workload/
Turnitin. (n.d.). Turnitin’s AI writing detection available now. Turnitin. Retrieved May 1, 2024, from https://www.turnitin.co.uk/solutions/topics/ai-writing/
University of Lincoln. (2024). Artificial Intelligence (AI) Detection in Turnitin. https://digitaleducation.lincoln.ac.uk/resources-hub/artificial-intelligence-ai-detection-in-turnitin/
UNESCO (2018). UNESCO ICT Competency Framework for Teachers, Version 3. United Nations Educational, Scientific and Cultural Organization.
UKESF (2023). Future engineering skills in the age of artificial intelligence. UK Electronics Skills Foundation. https://www.ukesf.org/wp-content/uploads/AI-report-interactive-March-2023.pdf
Webb, M. (2023a, January 26). Exploring the potential for bias in ChatGPT. JISC Artificial Intelligence. https://nationalcentreforai.jiscinvolve.org/wp/2023/01/26/exploring-the-potential-for-bias-in-chatgpt/
Webb, M. (2023b, September 18). AI Detection – Latest Recommendations. JISC Artificial Intelligence. https://nationalcentreforai.jiscinvolve.org/wp/2023/09/18/ai-detection-latest-recommendations/
Weber-Wulff, D., Ampjoma-Naumeca, A., Bjolobaba, S., Foltynek, T., Guerrero-Dib, J., Poppla, O., Sigut, P., & Waddington, L. (2023). Testing of Detection Tools for AI-Generated Text. Arxiv. https://arxiv.org/pdf/2306.15666.pdf
Zawacki-Richter, O., Marín, V. I., Bond, M., Gouverneur, F., & Hunger, I. (2019). Systematic review of research on artificial intelligence applications in higher education—where are the educators? International Journal of Educational Technology in Higher Education, 16(1), 39. https://doi.org/10.1186/s41239-019-0171-0
Appendix 1
Questions from first survey:
- From reading the information on the webpage, what do you hope that TeacherMatic will be able to help you with?
- How do you feel about using a tool such as TeacherMatic to support your work? Please include both positive and negative feelings where relevant.
- How do you think the student experience will be affected by your use of TeacherMatic? Please be as specific as possible.
- What do you think students will think of teachers using tools such as TeacherMatic
- What concerns do you have about using TeacherMatic? Please be as specific as you can.
Appendix 2
Questions from second survey:
- Do the documents make sense?
- Are they applicable to your context?
- What from the documents could/would you use?
- Are you aware of students using AI?
- Do you think AI is a threat to assessment integrity?
- Where are you on a continuum of enthusiast to sceptic?
Appendix 3
OpenAI Chat GPT Responses (OpenAI, 2024).
You
Explain the application and use of mechanical systems in food processing / manufacturing:
ChatGPT
ChatGPT
Mechanical systems play a crucial role in food processing and manufacturing by automating various tasks, improving efficiency, ensuring product quality, and maintaining safety standards. Here’s a breakdown of their application and use:
- Mixing and Blending: Mechanical mixers and blenders are employed to uniformly mix ingredients in food processing. Whether it’s mixing dough for bread or blending ingredients for sauces, mechanical systems ensure consistency and quality.
- Cutting and Slicing: Mechanical cutting and slicing machines are used to precisely cut fruits, vegetables, meats, and other food items to desired shapes and sizes. This ensures uniformity in the final product and increases production speed.
- Grinding and Milling: Machines like grinders and mills are utilized to grind grains, spices, and other ingredients into powders or pastes. They provide consistent texture and particle size, essential for various food products.
- Cooking and Heating: Mechanical systems such as ovens, steamers, and fryers are used for cooking and heating food items. These systems offer precise control over temperature and cooking time, ensuring food safety and quality.
- Packaging: Packaging machinery is employed to pack food products into containers, pouches, or bags. These machines can handle different packaging materials and ensure proper sealing to maintain freshness and extend shelf life.
- Sorting and Inspection: Mechanical sorting and inspection systems are used to remove defective or foreign materials from food products. These systems employ sensors, cameras, and automated mechanisms to detect and eliminate contaminants, ensuring product safety.
- Conveying and Transporting: Conveyor belts and automated transport systems are used to move raw materials and finished products throughout the processing plant. They facilitate smooth workflow, reduce manual handling, and minimize the risk of contamination.
- Cleaning and Sanitizing: Mechanical cleaning systems, such as industrial washers and sanitizers, are crucial for maintaining hygiene in food processing facilities. They ensure equipment and surfaces are thoroughly cleaned and sanitized to prevent foodborne illnesses.
- Quality Control: Mechanical systems are integrated with sensors and monitoring devices to continuously monitor various parameters such as temperature, pressure, and moisture levels during processing. This enables real-time quality control and ensures adherence to product specifications.
- Waste Management: Mechanical systems are also utilized for waste management in food processing plants. This includes equipment for recycling, composting, and waste disposal, helping facilities to minimize environmental impact and adhere to sustainability goals.
In summary, mechanical systems in food processing and manufacturing play a vital role in streamlining operations, ensuring product quality and safety, and meeting industry regulations and standards. They enable efficient, hygienic, and cost-effective production of a wide range of food products for consumers worldwide.
You
Produce a writing frame for a reflective account about the application and use of mechanical systems in food processing / manufacturing including: cams and followers, chain and belt drives, clutches and brakes, gears and gear drives, levers and linkage mechanisms, pumps, transmission shafts and valves
ChatGPT
ChatGPT
Title: Reflective Account: Application and Use of Mechanical Systems in Food Processing/Manufacturing
Introduction:
- Brief overview of the importance of mechanical systems in food processing/manufacturing.
- Introduction to the specific mechanical systems to be discussed: cams and followers, chain and belt drives, clutches and brakes, gears and gear drives, levers and linkage mechanisms, pumps, transmission shafts, and valves.
- Explanation of the purpose of this reflective account: to explore personal experiences and insights regarding the application and effectiveness of these mechanical systems in the food industry.
Body:
- Cams and Followers:
- Description of how cams and followers are utilized in food processing/manufacturing machinery.
- Personal experiences with machinery employing cams and followers.
- Reflection on the effectiveness and efficiency of cams and followers in specific applications within the food industry.
- Chain and Belt Drives:
- Explanation of the role of chain and belt drives in transferring power within food processing machinery.
- Personal anecdotes or observations related to the use of chain and belt drives.
- Reflection on the reliability and maintenance requirements of chain and belt drives in the food manufacturing environment.
- Clutches and Brakes:
- Overview of how clutches and brakes are applied in food processing equipment for control and safety.
- Personal instances where clutches and brakes have been pivotal in ensuring smooth operation or preventing accidents.
- Reflection on the importance of proper functioning and maintenance of clutches and brakes for operational efficiency and worker safety.
- Gears and Gear Drives:
- Description of the role of gears and gear drives in food processing machinery for speed reduction, torque amplification, and motion control.
- Personal experiences working with machinery incorporating gears and gear drives.
- Reflection on the advantages and limitations of gears and gear drives in food processing applications.
- Levers and Linkage Mechanisms:
- Explanation of how levers and linkage mechanisms are utilized for mechanical advantage and motion control in food processing equipment.
- Examples of specific applications of levers and linkage mechanisms in the food industry.
- Reflection on the versatility and effectiveness of levers and linkage mechanisms in optimizing processes and equipment design.
- Pumps:
- Overview of the importance of pumps in fluid handling and transfer within food processing facilities.
- Personal encounters with pump systems in the food manufacturing context.
- Reflection on the significance of pump reliability and efficiency for maintaining production continuity and product quality.
- Transmission Shafts:
- Description of the role of transmission shafts in transmitting power and rotational motion in food processing machinery.
- Personal observations regarding the use of transmission shafts in food manufacturing equipment.
- Reflection on the challenges and considerations associated with the design and maintenance of transmission shaft systems.
- Valves:
- Explanation of the function of valves in regulating flow, pressure, and direction of fluids in food processing operations.
- Personal instances where valves have been critical for process control and product quality assurance.
- Reflection on the importance of valve selection, positioning, and maintenance for efficient and hygienic food processing.
Conclusion:
- Summary of key insights gained from reflecting on the application and use of mechanical systems in food processing/manufacturing.
- Final thoughts on the significance of these mechanical components for ensuring efficiency, safety, and quality in the food industry.
- Consideration of potential areas for improvement or innovation in mechanical systems to address evolving challenges and demands in food processing.
References for Construct, Connect, Collaborate, Community.
Barrows, H. S. (1996). Problem-based learning in medicine and beyond: A brief overview. New Directions for Teaching and Learning, 1996(68), 3-12. https://doi.org/10.1002/tl.37219966804
Bayne, S., Evans, P., Ewins, R., Knox, J., Lamb, J., Macleod, H., O’Shea, C., Ross, J., Sheail, P., & Sinclair, C. (2020). The Manifesto for Teaching Online. The MIT Press. https://mitpress.mit.edu/9780262539838/
Bruner, J.S. (1960). The Process of Education. Harvard University Press. https://www.hup.harvard.edu/books/9780674710016
Carless, D. (2016). Feedback as Dialogue. In: Peters, M. (eds) Encyclopedia of Educational Philosophy and Theory. Springer. https://doi.org/10.1007/978-981-287-532-7_389-1
Danish, J. A., & Gresalfi, M. (2018). Cognitive and sociocultural perspectives on learning: Tensions and synergy in the learning sciences. In Fischer, F., Hmelo-Silver, C. E., Goldman, S. R., & Reimann, P. (Eds.), International handbook of the learning sciences (pp. 34-43). Routledge. https://doi.org/10.4324/9781315617572
Garrison, D., Anderson, T., & Archer, W. (1999). Critical Inquiry in a Text-Based Environment: Computer Conferencing in Higher Education. The Internet and Higher Education, 2, 87-105. https://doi.org/10.1016/S1096-7516(00)00016-6
Harasim, L. (2017). Learning Theory and Online Technologies (2nd ed.). Routledge. https://doi.org/10.4324/9781315716831
Hmelo-Silver, C., Duncan, R. G., & Chinn, C. A. (2007). Scaffolding and achievement in problem-based and inquiry learning: A response to Kirschner, Sweller, and. Educational Psychologist, 42(2), 99-107. https://doi.org/10.1080/00461520701263368
Kirschner, P.A., & Hendrick, C. (2020). How Learning Happens: Seminal Works in Educational Psychology and What They Mean in Practice (1st ed.). Routledge. https://doi.org/10.4324/9780429061523
Lave, J., & Wenger, E. (1991). Situated learning. Legitimate peripheral participation. Cambridge: University of Cambridge Press. https://doi.org/10.1017/CBO9780511815355
Peacock, S., Cowan, J., Irvine, L. & Williams, J. (2020). An Exploration Into the Importance of a Sense of Belonging for Online Learners. International Review of Research in Open and Distributed Learning, 21(2), 18–35. https://doi.org/10.19173/irrodl.v20i5.4539
Rakow, K. E., Upsher, R. J., Foster, J. L. H., Byrom, N. C., & Dommett, E. J. (2023). “It Ain’t What You Use, It’s the Way That You Use It”: How Virtual Learning Environments May Impact Student Mental Wellbeing. Education Sciences, 13(7), 749. https://doi.org/10.3390/educsci13070749
Ratniece, D. (2018). Cognitive development in active eLearning. International Journal of Engineering and Technology (UAE), 7(2), 53-57. https://doi.org/10.14419/ijet.v7i2.28.12881
Sankaranarayanan, R., Kwon, K., & Cho, Y. (2021). Exploring the differences between individuals and groups during the problem-solving process: The collective working-memory effect and the role of collaborative interactions. Journal of Interactive Learning Research. 32 (1), 43-66. https://www.learntechlib.org/primary/p/217515/
Siemens, G. (2005). Connectivism: A learning theory for the digital age. International Journal of Instructional Technology & Distance Learning, 2, 3-10. http://www.itdl.org/Journal/Jan_05/article01.htm
Stommel, J. (2018). Learning is not a mechanism. In An Urgency of teachers. https://pressbooks.pub/criticaldigitalpedagogy/chapter/learning-is-not-a-mechanism/
Susman, K. (2021). Between the tiles: The psychology of the virtual room. Appropriating and subverting the digital sphere for authentic and meaningful encounter. Person-Centered & Experiential Psychotherapies, 20(4), 327-344. https://doi.org/10.1080/14779757.2021.1938180
Sweller, J. (1988). Cognitive load during problem solving: Effects on learning. Cognitive Science, 12(2), 257–285. https://doi.org/10.1207/s15516709cog1202_4
Wenger, E. (1998). Communities of Practice: Learning, Meaning, and Identity. Cambridge University Press. https://doi.org/10.1017/CBO9780511803932
Wenger, E. (2000). Communities of Practice and Social Learning Systems. Organization, 7(2), 225-246. https://doi.org/10.1177/135050840072002
Wenger-Trayner, E. and Wenger-Trayner, B. (2015) An introduction to communities of practice: a brief overview of the concept and its uses. https://www.wenger-trayner.com/introduction-to-communities-of-practice
Towards a 21st century digital taxonomy for vocational education
“I’ve come up with a set of rules that describe our reactions to technologies,” writes the late, great, Douglas Adams in The Salmon of Doubt:
“1. Anything that is in the world when you’re born is normal and ordinary and is just a natural part of the way the world works.
2. Anything that’s invented between when you’re fifteen and thirty-five is new and exciting and revolutionary and you can probably get a career in it.
3. Anything invented after you’re thirty-five is against the natural order of things.”
I teach in the post-compulsory education sector in the UK, which comprises further education colleges, sixth form colleges, specialist colleges such as Leeds College of Building (LCB), and private training organisations. At LCB we provide high quality, specialist construction and building services engineering vocational education.
I teach electrical apprentices who attend as day-release students. My students age range is typically between the ages of 16 and 21. They usually the start on level three apprenticeship either straight from school or having done one year in college on a full-time level two electrical course whilst obtaining maths and English GCSEs which is an entry requirement to the apprenticeship program. However, I often have students in their 20s and 30s, and have even had a student in his 50s.
In this blog I’m going to discuss digital literacy – what has been written about it by other people, and how my apprentices use technology. I’m going to write about my own digital journey, and contrast it with that of my apprentices. I’ll consider what this means for my pedagogical approaches, and the importance of digital literacy for this group of learners.
Before I get into all that though, it is important to understand the world in which I teach. In future, electrical installations will consist of distributed local power generation. Devices, such as heaters, machinery, car charging and the like, will communicate with smart meters and use power, or in the case of batteries, charge when the price is very low, or free or the consumer will even be paid to take energy off the grid. This new landscape of 21st-century electrical installation will be smart and will require devices to be programmed. This will therefore require high levels of digital literacy in electricians to install the systems.
Previous iterations of the electrical apprenticeship (until 2018) included mandatory level two functional skills training in ICT, giving apprentices key digital skills enabling them to use these when constructing their online portfolios or just in the efficient use of Word, Google Chrome, or OneDrive (City & Guilds, 2018). Without this module, they would not have been allowed to progress to their endpoint assessment and fully qualify as electricians in England. Since 2018, however, this requirement has been removed from the apprenticeship standard. This is unfortunate as in the same period there has been the rapid adoption of smart technologies in the electrical industry. It is therefore essential that digital skills are included in electrical education.
Douglas Adams was hugely enthusiastic about technology, and his ‘Hitchhikers Guide to the Galaxy’ inspired early work on the internet. The quote I started with anticipates the thoughts of Marc Prensky (2001) in his influential work on Digital Natives and Digital Immigrants. Adams succinctly and humorously describes the situation in which many of us find ourselves – that is that if we’ve grown up with technology, we can cope, understand, and use it. However, after a certain age, we struggle to understand and use it.
Echoing Douglas Adams, Prensky suggests people can be classified as Digital Natives or Digital Immigrants based on their year of birth. After 1980 they are natives – people who have grown up with the technology in a media-rich world and instinctively know how to use it. Before 1980 they are immigrants – people who haven’t grown up with technology, were born before mass digital technology, have had the technology thrust on them and find it harder to accept and use new technology.
Prensky suggests that the native would be fluent in the digital languages of computers, video games and the internet. They should be better multitaskers, prefer digital communication – and as they have grown up with a digital world – the use of digital tools should be second nature to them. They should also prefer experiential learning rather than traditional passive learning. The immigrant, however, will use digital technology, but will not find it second nature. Digital immigrants must put in extra effort to use digital tools and they have a cautious approach to technology. They are less digitally intuitive. Traditional communication is preferred over digital, as are traditional forms of learning.
Children are naturally curious, (Piaget, 1964) and this can help them to learn to navigate digital tools. This natural curiosity, combined with ready access to digital tools through childhood could help children to develop as digital natives. Sugata Mitra conducted the famous Hole in the Wall study (2001) in which he placed a computer in the wall of a slum in Delhi. In this experiment he made the computer accessible only to children by the physical design of the access to the keyboard and monitor position. Mitra claims this experiment as a massive success, demonstrating what he calls Minimally Invasive Education where the children taught themselves. Others, however, have suggested he has exaggerated its success. As Warschauer, (2003) pointed out due to the lack of scaffolded learning this minimally invasive education only produced surface or shallow learning. In addition, it was noted that often the internet DSL link did not work, the equipment failed, and eventually the site was abandoned.
Most of my electrical apprentices should be Natives as they have grown up with technology and they should have the appropriate level of digital literacy to enable them to navigate through their apprenticeship. Why is the digital literacy of apprentices important? The possibilities that digital spaces also give the opportunity of creating virtual spaces of community and belonging. Vocational education happens both in the classroom and in the workplace. Students on vocational programs often only meet one day a week in person, however, by utilising the power of the classroom in their pocket an extension of the learning environment can be created. By moving all content and as much assessment as possible into the digital domain, a virtual college is available to them 24/7. Not only for accessing their theoretical work, or even the ability to easily gather evidence for their work portfolios, but also creating spaces where they can share information with others in their group. They can form active online communities between the lessons each week, removing the sense that they are only part of the group for one day a week. Checking up on each other, arranging social activities or just to check which room they will be in on any given week.
Sense of belonging is Important as it fosters better student wellbeing, together with better educational attainment (Korpershoek et al., 2020). The sense of relational belonging can be enhanced using digital tools which is especially important with day release students. Gravett et al. (2022) discusses this from the perspective of higher education students, but it can also be considered in the context of further education apprentices. Gravett et al. (2022) argue that the students of today experience their learning spaces very differently than did students in the past and that the use of digital spaces is now taken as an accepted and expected part of the student experience. Therefore, the fostering of belonging through our digital tools and spaces should be encouraged. Digital education offers the possibility of transformational change, connectedness, and unlimited access to learning materials (Haleem et al., 2022). To maximise these benefits, we need a better understanding of the relationship our students have with digital technology and how it differs from our own.
Throughout my life, I have been fascinated by technology. I recall spending time in the last year of primary school begging to be allowed to play with the school’s Research Machines 480z and trying to modify BASIC programs so that they would work on that machine. In secondary school, I joined the computer club and had exposure to Spectrums, Commodore 64s, and even a Sinclair QL – remember them? I was always taken with the possibilities that technology could offer, rather than just gaming like everyone around me. I was given a ZX81 for Christmas around the same time, with the wobbling RAM pack, and after spending time writing – well, rather copying – skiing games into the machine, I set about writing an information system for it. It would tell you the news, the weather, what’s on TV, etc. And I made something that was quite like teletext, and you could ask it what the weather was today – however, this being before the connection to the internet, I had to input the news and weather each day into the system.
When I first started secondary school in 1983, we had one computer in its own specialist room that you might be able to use if you were deemed able enough in maths. Through my time in secondary school, the one computer became a room of BBC Micros to finally, in the sixth form, Acorn Archimedes computers, and one Apple Macintosh 128. In the space of six years, my experience had gone from a command line to a graphic user interface, and I’m currently writing this blog on a MacBook.
This would indicate that Prensky would see me as an immigrant as I grew up during the rapid development of digital technologies rather than being “born digital” (Seely Brown, 2008). However, as someone who has grown up with computers and has used them creatively and professionally for the last 40 years, I think that the Digital Native vs. Digital Immigrant debate lacks nuance. Prensky is being incongruous as the whole modern computer industry was created by people born before 1980. Digital Native vs. Digital Immigrants does not reflect the rapid change of technology, or the relationship people have to it.
I see this digital divide through a different lens. I think that age doesn’t necessarily play a part in someone’s digital literacy. We all have different levels of experience of, and exposure to digital technology. Our skills are formed around this exposure and interaction with hardware or software. Furthermore, our motivations for using the technology will also impact our ability to engage with it (Dunn & Kennedy, 2019). In the context of observing my own students, I see this daily. If, as Prensky suggests, they should be digital natives, they should be able to navigate the digital world and have excellent digital literacy. However, they are consumers of technology. They consume YouTube, gaming sites, porn, news sites of dubious quality, and a large proportion of them consume the misinformation and misogyny of people like Andrew Tate. During their schooling, they haven’t been taught critical thinking to allow them to navigate the digital world (Polizzi, 2020). As educators it is our duty to counteract this. Indeed, our ETF professional standards for teachers within this sector state that we should “Select and use digital technologies safely and to promote learning” and “promote and support positive learner behaviour, attitudes and wellbeing.” (ETF, 2022) Thus, we should be at the forefront of giving our students the digital literacy, critical evaluation tools that they need to navigate their digital world.
This is what Paul Gilster argues in his book Digital Literacy. He states that there are a number of core competencies that must be acquired by someone so that they can successfully navigate through the internet-enabled world. He points out that being digitally literate goes beyond the ability to use a particular digital technology. Rather, he suggests that digital literacy is the ability to find and interpret information from a wide selection of digital sources and then to critically evaluate the information so that it can be used effectively. He believes that a digitally literate individual can take information from many different digital sources, synthesise it and then produce coherent information that can be communicated clearly. Digital literacy, for him, is the ability to understand information in the context of a digitally-enabled world, (Gilster, 1997)
However, the cohorts of apprentices I have encountered over the last four years do not display the level of digital literacy required as outlined by Gilster. They expect the technology to work and provide the solution, answer, or support for their work immediately and without them having to analyse the results given. They don’t seem to have the ability to use the discovery mode of learning and are afraid of exploring in case they ‘get it wrong’ or ‘break it’. I’m not sure where these responses come from; I think that maybe it’s a hangover from secondary education in the UK, which seems to have become more rigid and less based on discovery and more on exams and targets leaving less time for discovery or experimentation. They are also uncritical of the answers they are given by the technology. They will accept as fact what a quick Google search gives them. For example, every year I get the same answers on an electrical science worksheet to the question: What is a cell? Rather than giving an answer telling me about a device that produces electricity from a chemical reaction, they tell me about biological cells, membranes, and molecules as that is the first answer given by Google.
Gilster’s book was written when the world thought Apple was dead, before the return of Steve Jobs, and the iMac, iTunes, iPods, iPhones, iPads and the iCloud that dominate our world now, either in their Apple form or in the countless copies. Prensky was writing before the era of Facebook, X, TikTok and all the other social media sites that have infiltrated our world. Gilster didn’t anticipate the explosion of commercially driven internet sites where algorithms drive consumption. My learners don’t need to be explorers to engage with content, which in turn reduces their digital skills. However, Gilster’s ideas are still relevant as he states that we need to adapt our skills as the digital technologies change.
Having considered the digital landscape over the last half century, together with my own story and observing my apprentices over the last four years, I feel that Prensky’s descriptions need revising and expanding. We need a better range of descriptors to illustrate the variation in digital literacies, one that can encompass multiple identities to better understand our students, and thus develop the digital literacy of our students. Therefore, it would be better to look at the divide through the following lenses:
- A digital consumer – someone who consumes digital content.
- A digital creator – someone who creates digital content.
- A digital explorer – someone who is curious, questioning and investigates digital content.
- A digital exile – excluded from digital due to poverty, lack of access or lack of skill.
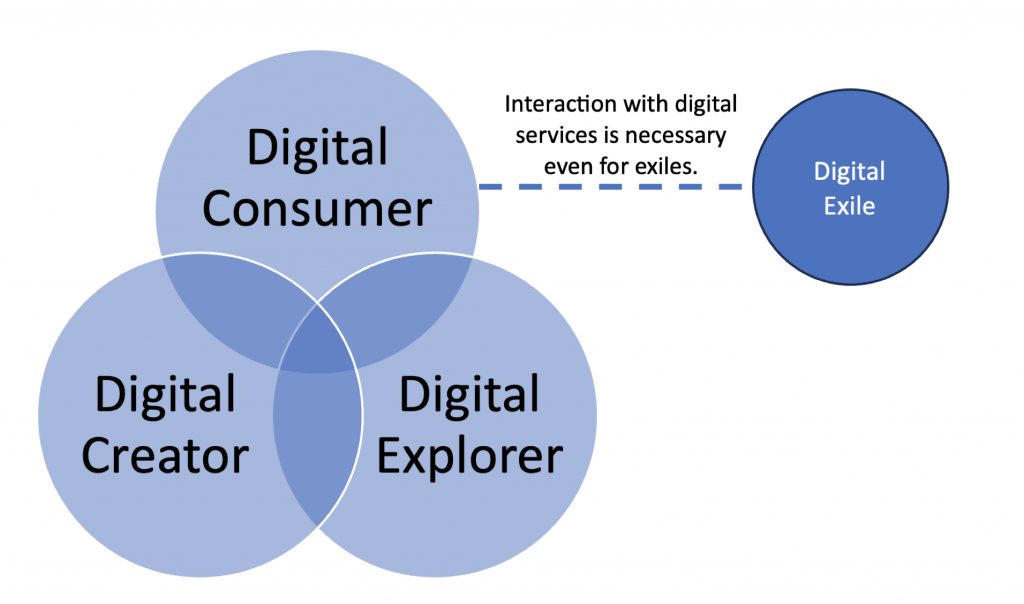
These identifiers do not have to be as rigid as in the immigrants vs. natives descriptors. One can be simultaneously a consumer and creator: for example, when creating a TikTok video, or a creator and explorer when coding new content. Equally an exile having little or no digital literacy must be a consumer at times – when accessing online services – which brings into the question of digital inequality. Unequal access to digital technology and services is something to consider in the context of other educational settings. However, my students are employed, have computing technology in their pocket (which sometimes I wish they would stop using in the classroom!) and have access to computer-enhanced classrooms when in college. This is a fortunate situation to be in and removes the worry of digital exclusion due to poverty when introducing more digital tools to the classroom. However, there could still be a level of digital exclusion due to lack of skills.
I have observed the use of digital technology by my students, and they fit into these new digital identity descriptors well. Most of them are digital consumers. They lack a lot of the necessary digital literacy as outlined by Gilster. There is a deficit in their training considering the subject they are studying and the needs of the electrical industry. They need to develop their digital skills to be creators, consumers, and explorers.
I use technology to scaffold the learning of my students. Using MS Teams to scaffold their learning in conjunction with the principles of Problem-Based Learning (PBL). PBL’s constructivist roots promote active learning with student ownership where knowledge is constructed through experience and social interaction (Barrows, 1996). Digital brings the social interaction, especially when leaners are not in college, and enables me to support their learning. This scaffolded PBL approach has been shown to reduce cognitive load, allowing for deep learning (Hmelo-Silver et al. 2007). I use MS Teams as the course repository for all the information they need for each module. Each subject or unit gets its own channel, and within that channel, I use the announcement function to break up each topic. I also use good background graphics on each header so that when the students are looking for each subject, they have a visual clue which aids in identifying the topic. I also check that it looks good and works well on phones as this is the access point my students have when not in college.
I also use Microsoft Forms to create timed multiple choice questions (MCQs) for the students which mirror the actual assessment that they will do – these can then be set as assignments in MS Teams and I can monitor the students while they are completing the tasks – it also enables me to monitor the answers and get an overall picture of which questions the cohort finds easy and which ones have been more problematic.
This data enables me to then target my teaching, using the principles of the spiral curriculum (Bruner, 1960) to bring back those subjects that need further work in future sessions. As their assessments for each module include an MCQ, this is a valid and authentic way of using digital pedagogy in formative assessments, modelling their final summative ones.
I try to make teaching spaces of psychological safety where students can ask questions, make mistakes, and ask for support, which in turn promotes deeper learning and where more demanding work can take place (Hughes et al., 2023). This approach enables my students to experiment with technology and learn to become Digital Explorers and develop their connectivism where they interact with distributed sources of information and make learning networks (Siemens, 2005).
The new landscape of digital technology within education, which is being encouraged by government in policy and funding – at LCB we have just received part of a £6.9 million funding package from government for the development of digital teaching – presents several challenges such as how to integrate the technology into the existing curriculum However, we must remember that good digital pedagogy is good pedagogy (Stommel, 2018), and we shouldn’t accept the technology just because it is new. We should critically evaluate the pedagogical usefulness of the digital tool we wish to use, just as we would evaluate our own pedagogy depending on our context and subject specialism. We must on the one hand prepare our students for the digital future that they will encounter (Iivari et al., 2020), whilst at the same time upskilling our own digital literacies. In some situations, a Kahoot quiz makes an excellent replacement for mini whiteboards, whereas in other situations the use of self-marking MS Forms set as assignments in MS Teams is appropriate. The tools shouldn’t be used just because they are available. Rather, they should be used to enhance the learning process. If they don’t bring anything useful to the party, then what’s the point?
Teachers in the 21st century need a high level of digital competence. Indeed, there is an expectation from government that teachers will have at least a level one standard of digital literacy with the teaching of this embedded within their initial teacher training (Department for Education, 2023). Furthermore, without a high level of digital competence then the opportunities and enhanced learning outcomes that digital technology offer cannot be achieved.
Fortunately, there are a number of frameworks that can be deployed to help the teacher frame and improve their digital literacy skills, integrating digital technology with teaching. These are: the European Framework for the Digital Competence of Educators (DigCompEu), UNESCO ICT Competency Framework (ICT CFT), Technological Pedagogical Content Knowledge (TPACK) and the SAMR Framework for Technology Integration (Bećirović, 2023).
TPACK is a framework that allows teachers think about the challenges in applying digital technologies in their teaching (Koehler & Mishra, 2009). As it focuses on the intersection of technology, pedagogy, and content knowledge (Koehler et al., 2013) it is my preference as a framework in which to consider the effectiveness of digital tools within my teaching. TPACK’s strong focus on integrating technology with technological knowledge is appropriate in teaching electrical apprentices due to the nature of electrical apprenticeship and the availability of digital tools. It allows for the complex nature of my discipline and acknowledges the “interweaving of many kinds of specialist knowledge” (Koehler & Mishra, 2009). The focus on problem solving, group work, and digital integration is especially useful as it matches my subject specific pedagogy. TPACK offers a way of measuring my progress and effectiveness of digitally transforming the curriculum and, as Koehler et al. (2013) point out, “move beyond oversimplified approaches that treat technology as an ‘add on’”.
Another consideration when integrating digital technology within teaching practice is the availability and quality of the IT and the availability of IT support. As we have seen with the Hole in the Wall project without sufficient IT support and resources the project fails or gets abandoned. Likewise, within our own settings we need IT systems that work if we are going to deeply embed digital learning. For example, it is quite common that IT support and infrastructure are behind the curve of digital pedagogical development and that networks within schools and colleges are not stable or fast enough to support the widespread digitisation of the curriculum. Or it might be simply the case that the mobile laptop trolley is never plugged in to charge overnight. IT support need to work together with practitioners allowing the experimentation with new digital tools rather than frustrate them with endless bureaucratic systems that make the implementation of new tools difficult or impossible. Decision makers and managers need to ensure that IT departments work with practitioners in enabling the development of digital tools based on good digital pedagogy enabling our students to have high class, quality digital education.
All of the above may well be true but the whole world is about to change and as Dickinson (2023) points out, AI is the “avalanche that is about to hit us”. It is going to be built into everything and as AI detectors do not work (Weber-Wulff et al., 2023) we need to rethink our pedagogy and assessment strategies (Lambert & Stevens, 2023) to take account of this. The old world is over now that ChatGPT4 can analyse your own writing and generate text in your style. Digital pedagogy needs to embrace an appropriate use of AI along with all the other digital tools. I find it equally exciting and scary so I’m leaving the last words to Douglas Adams: “Don’t Panic”.
References:
Adams, D, (1979). The Hitchhiker’s Guide to the Galaxy. Pan Books
Adams, D (2002). The Salmon of Doubt: Hitchhiking the Galaxy One Last Time. Macmillan
Barrows, H. S. (1996). Problem-based learning in medicine and beyond: A brief overview. New Directions for Teaching and Learning. 1996 (68), 3–12. https://doi.org/10.1002/tl.37219966804.
Bećirović, S. (2023). Fostering Digital Competence in Teachers: A Review of Existing Frameworks. In: Digital Pedagogy. SpringerBriefs in Education. Springer. https://doi.org/10.1007/978-981-99-0444-0_5
Bruner, J.S. (1960). The Process of Education. Harvard University Press
City & Guilds (2018). City & Guilds Level 3 NVQ Diplomas in Electrotechnical Technology (2357) Qualification handbook. City & Guilds.
Department for Education. (2023) Overview: the initial teacher education system for FE. https://www.gov.uk/government/publications/further-education-initial-teacher-education/overview-the-initial-teacher-education-system-for-fe
Dickinson, J. (2023, March 17). An avalanche really is coming this time. WonkHE. https://wonkhe.com/blogs/an-avalanche-really-is-coming-this-time/
Dunn, T. J., & Kennedy, M. (2019). Technology Enhanced Learning in higher education; motivations, engagement and academic achievement. Computers & Education 137. 104-113. https://doi.org/10.1016/j.compedu.2019.04.004
Education and Teaching Foundation (2022). Professional Standards. Retrieved 21st October, 2023, from https://www.et-foundation.co.uk/wp-content/uploads/2022/04/PS-for-Teachers_Summary-of-Standards_A4-Poster_Final.pdf
Gilster, P. (1997). Digital Literacy. Wiley Computer Pub.
Gravett, K., Baughan, P., Rao, N. et al. Spaces and Places for Connection in the Postdigital University. Postdigit Sci Educ 5, 694–715 (2023). https://doi.org/10.1007/s42438-022-00317-0
Haleem, A., Javaid, M., Qadri, M. A., & Suman, R. (2022). Understanding the role of digital technologies in education: A review. Sustainable operations and Computers, 3, 275 – 285 https://doi.org/10.1016/j.susoc.2022.05.004
Hmelo-Silver, C., Duncan, R. G., & Chinn, C. A. (2007). Scaffolding and achievement in problem-based and inquiry learning: A response to Kirschner, Sweller, and. Educational Psychologist, 42(2), 99-107. https://doi.org/10.1080/00461520701263368
Hughes, G., Upsher, R., Nobili, A., Kirkman, A., Wilson, C., Bowers-Brown, T., Foster, J., Bradley, S., & Byrom, N. (2022). Education for mental health: Enhancing student mental health through curriculum and pedagogy. Advance HE. https://s3.eu-west-2.amazonaws.com/assets.creode.advancehe-document-manager/documents/advance-he/AdvHE_Education%20for%20mental%20health_online_1644243779.pdf
Iivari N, Sharma S, Ventä-Olkkonen L (2020) Digital transformation of everyday life–how COVID-19 pandemic transformed the basic education of the young generation and why information management research should care? Int J Inf Manag 55:102183. https://doi.org/10.1016/j.ijinfomgt.2020.102183
Koehler, M. J., & Mishra, P. (2009). What is technological pedagogical content knowledge? Contemporary Issues in Technology and Teacher Education, 9(1). https://citejournal.org/volume-9/issue-1-09/general/what-is-technological-pedagogicalcontent-knowledge
Koehler, M. J., Mishra, P., & Cain, W. (2013). What is Technological Pedagogical Content Knowledge (TPACK)? Journal of Education, 193(3), 13-19. https://doi.org/10.1177/002205741319300303
Korpershoek, H., Canrinus, E.T., Fokkens-Bruinsma M., & de Boer, H. (2020) The relationships between school belonging and students’ motivational, social-emotional, behavioural, and academic outcomes in secondary education: a meta-analytic review, Research Papers in Education, 35:6, 641-680, DOI: 10.1080/02671522.2019.1615116
Lambert, J., & Stevens, M. (2023) ChatGPT and Generative AI Technology: A Mixed Bag of Concerns and New Opportunities, Computers in the Schools, DOI: 10.1080/07380569.2023.2256710
Mitra, S., & Rana, V. (2001). Children and the Internet: Experiments with minimally invasive education in India. British Journal of Educational Technology, 32 (2), 221-232. https://doi.org/10.1111/1467-8535.00192
Piaget, J. (1964). Cognitive Development in Children: Development and Learning. Journal of Research in Science Teaching, 2, 176-186. http://dx.doi.org/10.1002/tea.3660020306
Polizzi, G. (2020). Digital literacy and the national curriculum for England: Learning from how the experts engage with and evaluate online content. Computers & Education 152. https://doi.org/10.1016/j.compedu.2020.103859
Prensky, M. (2001). Digital Natives, Digital Immigrants. MCB University Press, 9(5). https://doi.org/10.1108/10748120110424816
Seely-Brown, J. (2008), “Foreword”, in Iiyoshi, T. and Kumar, M. (Eds), Opening up Education, MIT Press https://mitp-content-server.mit.edu/books/content/sectbyfn/books_pres_0/7641/7641.pdf?dl=1
Siemens, G. (2005). Connectivism: A learning theory for the digital age. International Journal of Instructional Technology & Distance Learning, 2, 3-10. http://www.itdl.org/Journal/Jan_05/article01.htm
Stommel, J. (2018). Learning is not a mechanism. In An Urgency of teachers. https://pressbooks.pub/criticaldigitalpedagogy/chapter/learning-is-not-a-mechanism/
Warschauer, M (2003). Technology and Social Inclusion: Rethinking the Digital Divide. MIT Press. https://doi.org/10.7551/mitpress/6699.001.0001
Weber-Wulff, D., Ampjoma-Naumeca, A., Bjolobaba, S., Foltynek, T., Guerrero-Dib, J., Poppla, O., Sigut, P., & Waddington, L. (2023). Testing of Detection Tools for AI-Generated Text. Arxiv. https://arxiv.org/pdf/2306.15666.pdf